AI and Predictive Analytics: A Fraud Detection Dream Team
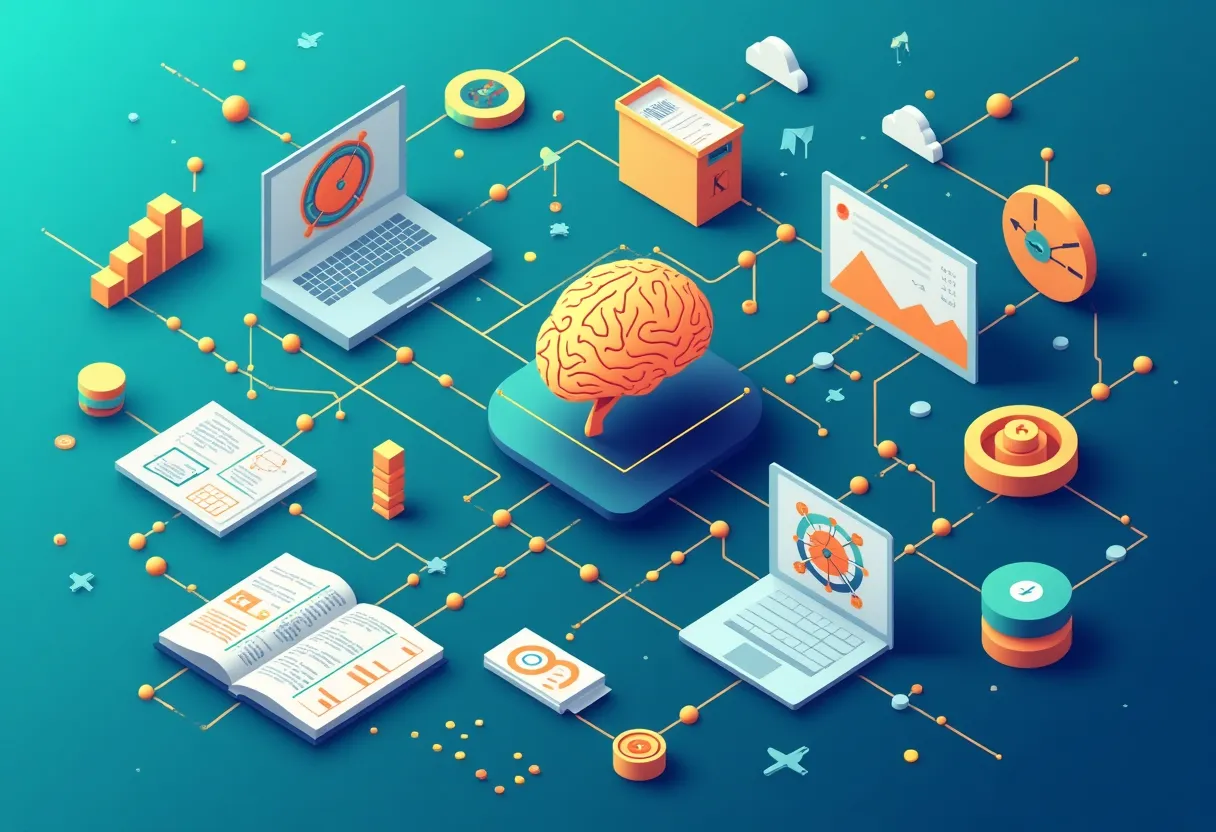
Introduction
Fraud detection is a significant challenge for the insurance industry, costing billions of dollars annually. Insurers must continually refine their strategies to prevent, detect, and mitigate fraud effectively. With the rise of sophisticated scammers and innovations in technology, traditional methods often fall short. This is where the powerful combination of artificial intelligence (AI) and predictive analytics comes into play. By leveraging these advanced technologies, insurers can enhance their ability to identify and prevent fraud in real-time, ultimately safeguarding their assets and maintaining customer trust.
Timely identification and prevention of fraud are critical not only for financial stability but also for improving the customer experience. The insurance landscape is changing rapidly; consumers expect swift service without compromising security. AI and predictive analytics are transforming the fraud detection process, enabling insurers to stay ahead of the fraud curve while enhancing their operational efficiency.
What is the Role of AI in Insurance Fraud Detection?
AI plays a transformative role in enhancing fraud detection mechanisms within the insurance sector. At its core, AI enhances data analysis by processing vast amounts of data at an unprecedented speed. This enables insurers to identify anomalies and red flags in claims that may indicate fraudulent activities. The integration of AI technologies, such as machine learning, allows insurers to continuously improve their data analysis capabilities, leading to more accurate fraud detection outcomes.
How does AI enhance data analysis in fraud detection?
AI enhances data analysis by automating the identification process of potential fraud through advanced algorithms that can analyze patterns and identify discrepancies in data sets. Unlike manual methods, which are often prone to human error, AI systems can continuously learn from past instances of fraud, adapting their detection techniques accordingly. For instance, if a particular type of fraud becomes prevalent, AI can adjust its analytical models in real-time to incorporate new variables, thus improving detection accuracy.
What machine learning techniques are commonly used in fraud detection?
Common machine learning techniques used in fraud detection include supervised learning, unsupervised learning, and reinforcement learning. Supervised learning involves training models on historical data labeled with known outcomes—this allows algorithms to learn patterns associated with both legitimate and fraudulent claims. Unsupervised learning, on the other hand, identifies hidden patterns in data without prior labeling, making it effective in discovering new types of fraud. Reinforcement learning allows models to learn from their own performance and make predictive adjustments, continually refining their accuracy.
How can AI algorithms adapt to emerging fraud patterns?
AI algorithms can adapt to emerging fraud patterns through continuous learning processes. As new fraud tactics develop, AI systems analyze the factors contributing to these changes and adjust their behavior accordingly. This adaptability stems from their intrinsic design, which thrives on exposure to diverse datasets. By regularly updating models with fresh data, insurers can not only recognize existing threats but also pre-emptively identify potential future threats, maintaining a proactive stance against fraud.
Why is Predictive Analytics Crucial for Insurance Fraud Detection?
Predictive analytics is a vital tool in the fight against insurance fraud, providing insurers with the capability to anticipate and mitigate risks before they materialize. It utilizes historical data to create models that predict future outcomes, allowing companies to focus their resources on the highest-risk areas. By leveraging predictive analytics, insurers can prioritize claims for review based on their calculated risk levels, streamlining the investigation process.
What is predictive analytics and how does it work in insurance?
Predictive analytics involves statistical techniques including data mining, predictive modeling, and machine learning to analyze historical and current data to identify patterns and gauge future outcomes. In insurance, this means using available data from claims, customer profiles, and external data sources to create risk profiles for new claims. For instance, if past data indicates that certain combinations of claims parameters are frequently associated with fraud, predictive models can flag new claims meeting those criteria for further scrutiny.
How can predictive modeling identify potential fraud risk?
Predictive modeling utilizes algorithms to process large volumes of data, identifying correlations and trends that may indicate fraudulent activity. By constructing models based on various attributes of claims—such as geographical location, claim amount, and claimant demographic—predictive analytics can assign risk scores to claims, allowing insurers to focus on those with the highest likelihood of fraud. This proactive identification enables quicker resolutions and significantly reduces financial losses.
What are the benefits of using predictive analytics over traditional methods?
Compared to traditional fraud detection methods, predictive analytics offers several distinct advantages. First, it allows for a more data-driven approach, grounding decisions in empirical evidence rather than intuition. This leads to improved accuracy in identifying fraud. Additionally, predictive analytics can analyze vast, complex datasets that would be impractical for manual reviews. This capability results in faster processing times and enhanced operational efficiency, empowering insurers to respond to potential fraud incidents promptly.
How do AI and Predictive Analytics Work Together in Fraud Detection?
The synergy between AI and predictive analytics creates a formidable defense against insurance fraud, enhancing overall effectiveness. AI's capacity to automate data analysis and predictive analytics’ ability to model potential outcomes collaborates to provide a comprehensive approach to identifying fraud. Together, these technologies foster a deeper understanding of patterns and trends, which can be leveraged to inform strategic decision-making.
In what ways do AI and predictive analytics complement each other?
AI and predictive analytics complement each other in several key areas. AI enhances the analytical capabilities of predictive models by applying machine learning algorithms that can sift through data and recognize trends that might not be apparent to human analysts. Conversely, predictive analytics informs AI systems by providing validated insights that can reinforce the algorithms’ learning processes, ultimately improving their predictive power. This collaboration enables a more adaptive and forward-looking fraud detection strategy.
What is the data flow between AI systems and predictive analytics models?
The data flow between AI systems and predictive analytics models involves a continuous loop of data input, analysis, and output. Initially, data collected from various sources is processed by AI systems to identify patterns and anomalies. Insights gleaned from this analysis are then fed back into predictive models, which refine their algorithms based on new developments in the data. This cycle repeats, continuously enhancing the models and their predictive capabilities.
How does the integration of AI enhance predictive analytics for fraud detection?
The integration of AI enhances predictive analytics by enabling real-time data processing and analysis. Traditional predictive analytics often rely on historical data snapshots for model development, while AI allows for the ongoing incorporation of new data. This reduces the lag time between fraud patterns emerging and detection, empowering insurers to respond more rapidly and effectively. By using AI to complement predictive analytics, insurers can build adaptive fraud detection systems that remain effective against evolving threats.
What Challenges Are Associated with Implementing AI and Predictive Analytics in Fraud Detection?
While AI and predictive analytics provide robust tools for combating insurance fraud, their implementation is not without challenges. Insurers often face hurdles related to technology adoption, data quality, and ethical considerations that can impede the effectiveness of these solutions.
What are the common hurdles insurers face in technology adoption?
Common hurdles insurance companies face when adopting AI and predictive analytics include resistance to change within the organizational culture, inadequacies in current technology infrastructure, and a shortage of skilled data scientists. Resistance to change can stem from a lack of understanding of the potential benefits that these technologies can bring. Furthermore, insurers may struggle with outdated legacy systems that are incompatible with advanced analytics tools, making integration difficult.
How can data quality impact fraud detection efforts?
Data quality is essential for effective fraud detection. Inaccurate, incomplete, or outdated data can lead to misguided analyses and flawed conclusions, ultimately resulting in missed fraud opportunities or false positives. Insurers must prioritize data cleansing and enrichment to ensure they have reliable datasets for AI and predictive analytics to operate effectively. Establishing robust data governance practices can help maintain data integrity and improve overall fraud detection accuracy.
What ethical considerations should insurers keep in mind?
When implementing AI and predictive analytics, ethical considerations are paramount. Insurers must ensure that their fraud detection processes do not unfairly target specific demographics or create biases that could lead to discriminatory practices. Moreover, transparency in how data is used and how decisions are made is important for maintaining customer trust. Insurers should establish ethical guidelines to address potential biases in data collection and analysis to protect consumers’ rights while leveraging powerful technologies.
What Success Stories Demonstrate the Effectiveness of AI and Predictive Analytics in Fraud Detection?
Numerous success stories illustrate how the integration of AI and predictive analytics can significantly enhance fraud detection efforts. These case studies underscore the tangible benefits that these technologies offer in protecting insurer’s assets and improving operational efficiencies.
What case studies show successful fraud prevention utilizing these technologies?
Case studies from leading insurance companies reveal that those employing AI and predictive analytics have seen measurable improvements in their fraud detection rates. For instance, several insurers have reported reductions in fraudulent claims by implementing machine learning algorithms that can quickly analyze and flag suspicious activities. Other insurers have experienced improved loss ratios and enhanced profitability through predictive models that intelligently filter fraudulent claims before payment.
What metrics are used to measure success in fraud detection initiatives?
Success in fraud detection initiatives can be measured through several key metrics, including reduction in fraudulent claims, overall loss ratios, and operational cost savings. Additionally, insurers may look at the speed at which claims can be processed legitimately—those utilizing AI and predictive analytics often experience significant improvements in processing times, enabling faster responses to customers while sifting out fraudulent claims efficiently.
How can lessons from these success stories guide future efforts?
The success stories of others can provide valuable insights for insurers looking to enhance their fraud detection capabilities. By analyzing what strategies have worked for others, insurers can adopt best practices and avoid common pitfalls. Continuous learning and adaptation based on industry success can position insurers ahead of emerging threats and improve their overall operational efficiency.
How Can Insurers Prepare for the Future of Fraud Detection with AI and Predictive Analytics?
As the landscape of insurance fraud evolves, so must the strategies employed by insurers. Organizations need to take proactive steps to implement AI and predictive analytics effectively, ensuring they are well-prepared for future developments in fraud detection.
What steps should organizations take to implement these technologies?
To implement AI and predictive analytics, organizations should conduct thorough assessments of their existing technology infrastructure and identify necessary upgrades. Collaboration between IT departments and data science teams is crucial to developing and deploy predictive models efficiently. Additionally, investing in training programs will help build a skilled workforce capable of leveraging these technologies effectively.
How can continuous learning and model refinement enhance fraud detection?
Continuous learning and model refinement are vital for staying relevant in the fraud detection arena. As fraud tactics evolve, the parameters of existing models must be adjusted to maintain effectiveness. Regularly updating the datasets used for training algorithms helps ensure that models remain responsive to new fraud techniques. Insurers must foster an environment of continuous improvement, where data scientists can iterate and enhance their models based on the latest trends.
What trends should insurers watch for in the evolving landscape of fraud detection?
Insurers should keep an eye on trends such as the increasing use of real-time data analysis, enhanced customer interactions through AI-driven systems, and the rise in machine learning capabilities. As technology advances, insurers who embrace these trends are likely to stay several steps ahead of fraudulent activities, enhancing their competitive advantage in the market. Furthermore, as regulatory scrutiny around data privacy increases, insurers must stay compliant while leveraging AI and analytics for fraud detection.
Conclusion
The transformative potential of AI and predictive analytics in insurance fraud detection cannot be overstated. These technologies allow insurers to proactively mitigate risks, improve their response times, and enhance overall operational efficiency. By embracing AI and predictive analytics, insurance companies can build a robust defense against evolving fraudulent behaviors and maintain consumer trust in an increasingly competitive landscape.
For organizations looking to further their understanding of predictive analytics, consider exploring our insights on the role of predictive analytics in preventing insurance fraud. If you're keen on implementing these technologies and want personalized guidance, contact us today.