AI-Driven Fraud Detection in Claims Automation
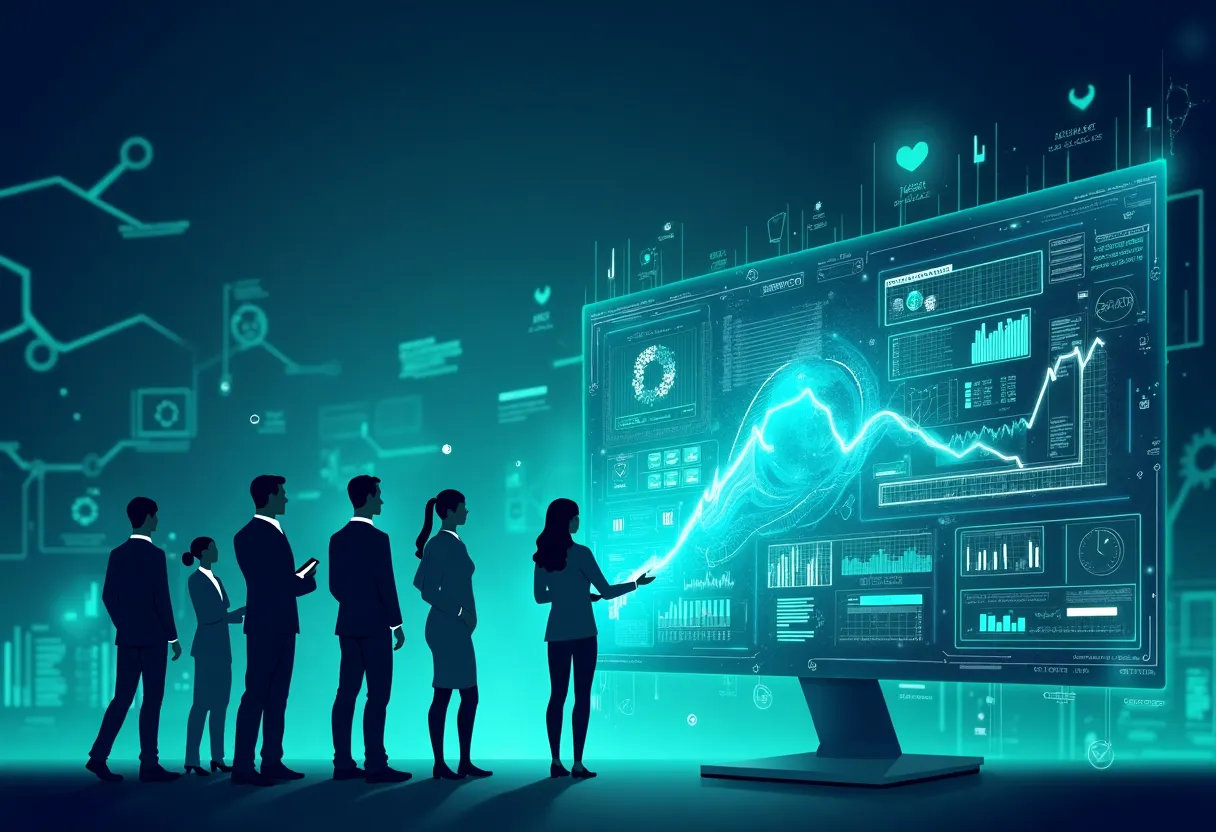
Introduction
Fraud in insurance claims poses a significant challenge to the industry, costing insurers millions each year and eroding customer trust. As a response, many companies are turning toward advanced technology solutions to combat these deceptive practices. Integrating artificial intelligence (AI) into claims automation is pivotal, offering enhanced efficiency and accuracy that traditional methods struggle to match. This blog will explore how AI enhances fraud detection, the types of fraud it can help identify, key components of AI-driven systems, measurement of effectiveness, and essential ethical considerations.
How Does AI Enhance Fraud Detection in Claims Automation?
Understanding AI’s Role in Claims Processing
Artificial intelligence in insurance refers to intelligent systems equipped to analyze large datasets and execute tasks traditionally performed by human agents. In claims processing, AI utilizes techniques such as machine learning (ML) and natural language processing (NLP) to automate and enhance various stages, from initial submission to final settlement.
Machine learning enables systems to recognize patterns in historical claims data, allowing them to identify anomalies indicative of fraudulent activities. Meanwhile, natural language processing aids in analyzing written claims data and communications, extracting relevant information that could signal potential fraud. Together, these technologies provide a robust framework for automating claims processing in a way that traditional methods simply cannot achieve.
Beyond Traditional Methods: The Advantages of AI
Traditional fraud detection relies heavily on established procedures and human judgment, which can be slow and prone to errors. AI-driven approaches revolutionize this landscape by providing several key advantages. For starters, the speed at which AI can analyze data dramatically surpasses conventional manual practices. As a result, claims can be processed quicker, ensuring faster resolutions for genuine claims while identifying fraudulent ones at an earlier stage.
Moreover, AI's accuracy is a game-changer. With sophisticated algorithms designed to learn from both historical data and emerging trends, AI systems are continually evolving to detect more complex fraud schemes. This capability not only leads to improved detection rates but also allows insurance providers to refine their overall risk assessment processes.
Real-World Applications of AI in Fraud Detection
AI's adoption in the insurance industry has already yielded impressive results, particularly in fraud detection. Numerous insurers have reported significant decreases in fraudulent claims through the implementation of AI-driven technologies. For example, a major insurance carrier utilized machine learning algorithms to analyze their claims data against known fraud patterns, resulting in a 40% increase in fraud detection accuracy compared to traditional methods. Metrics like reduced claim processing times and lower loss ratios further underscore the effectiveness of AI in identifying and mitigating fraud.
What Types of Fraud Can AI Help Identify in Claims?
Common Fraudulent Behaviors Targeted by AI
The landscape of insurance fraud is diverse, encompassing various schemes that can impact the bottom line of insurance companies. Some common fraudulent behaviors include staged accidents, where policyholders orchestrate collisions to claim compensation; inflated claims, which involve exaggerating the value of a genuine claim; and ghost policies, where individuals manipulate details to create fraudulent coverage.
AI models can efficiently pinpoint these activities by analyzing behavioral patterns and spotting inconsistencies in claims data. For instance, they might flag a claim where the circumstances described in the submission do not align with the historical behavior of similar claims, indicating possible fraud.
Anomaly Detection: How AI Spotlights Suspicious Patterns
One of AI's most powerful capabilities lies in its anomaly detection abilities. In this context, anomaly detection refers to identifying data points or patterns that deviate significantly from the norm. AI leverages complex algorithms to monitor claims in real-time, comparing each submission to historical data and known patterns of legitimate claims.
For example, if a claim is submitted for repair costs that are disproportionately high for the given incident type, the AI will flag it for further investigation. This capability allows for the identification of suspicious patterns, ensuring that potential fraud does not go unnoticed.
Continuous Learning: Adapting to New Fraud Techniques
The dynamic nature of fraud means that schemes are constantly evolving, but AI systems are designed to keep pace. Machine learning models continuously learn from new data, adapting to emerging fraud techniques. As fraudsters develop more sophisticated methods, so too do AI algorithms, enhancing their ability to identify and counteract new fraudulent behaviors.
Staying ahead of fraudulent trends is critical for insurance providers, and the adaptive capabilities of AI ensure that they can respond to changing landscapes effectively. This continuous learning not only bolsters accuracy over time but also instills greater confidence in the overall claims processing framework.
What Are the Key Components of AI-Driven Fraud Detection Systems?
Data Collection: The Foundation of Effective Fraud Detection
An essential facet of an AI-driven fraud detection system is robust data collection. The effectiveness of AI models hinges on the quality and comprehensiveness of data available for analysis. Insurers need to gather a vast array of data, including claims history, customer behavior, and even external data sources such as social media activity.
Comprehensive data enables a deeper understanding of customer behaviors and improves the model's ability to identify discrepancies that may suggest fraud. Therefore, building a solid foundation of diverse data is paramount for effective fraud detection.
Machine Learning Algorithms: The Backbone of Detection
The use of machine learning algorithms is integral to the functionality of AI-driven fraud detection systems. Various algorithms can be employed, including decision trees, neural networks, and ensemble methods, each tailored to specific data characteristics and requirements. Selecting the appropriate algorithm based on the type of data, volume, and complexity is critical for maximizing detection accuracy and operational efficiency.
As these algorithms assess incoming claims data, they evaluate risk levels and categorize claims as potentially fraudulent or legitimate based on established criteria. This classification process is fundamental in prioritizing claims for further investigation and ensuring that genuine claims are processed expeditiously.
Integration with Existing Claims Automation Systems
For AI to realize its full potential in fraud detection, it must be integrated seamlessly with existing claims automation systems. This integration entails aligning AI capabilities with current workflows while ensuring compatibility with legacy systems. Challenges might arise during integration, such as data silos and interoperability issues; however, solutions, such as APIs and cloud-based platforms, can facilitate smooth transitions.
When executed successfully, the integration of AI within existing frameworks not only enhances fraud detection but also streamlines the entire claims process, providing a holistic view of claims management.
How Can Insurers Measure the Effectiveness of AI-Driven Fraud Detection?
Key Performance Indicators (KPIs) to Monitor
To gauge the effectiveness of AI-driven fraud detection systems, insurers must monitor key performance indicators (KPIs). Suggested KPIs include the detection rate of fraudulent claims, the time taken to identify potential fraud, the ratio of false positives, and the overall loss ratio related to fraud.
These metrics are instrumental in assessing performance and ensure that systems are working efficiently to detect fraud while minimizing disruptions in legitimate claims processing. Maintaining a balance between thorough fraud detection and quick claim resolutions is essential for optimal operational efficacy.
Reporting and Analytics: Gaining Insights from Data
Robust reporting and analytics tools are crucial for tracking the success of fraud detection efforts. Insurers benefit from visualization techniques that showcase data trends and outcomes in an easily digestible format. By analyzing these outputs, stakeholders can glean insights into both successful fraud detection initiatives and areas requiring improvement.
Effective use of analytics empowers insurers to refine their fraud detection strategies continuously, ensuring a proactive rather than reactive approach to fraud prevention.
Feedback Loops: Using Results to Refine AI Systems
Implementing feedback loops is vital for the continuous improvement of AI algorithms. By collecting data on detection efficacy and analyzing the results, insurers can refine their systems and adapt their fraud detection strategies. Real-time data plays a crucial role in this process; it enables models to learn from past mistakes and successes alike, fostering a cycle of ongoing enhancement.
What Are the Ethical Considerations in AI-Driven Fraud Detection?
Data Privacy Concerns
As insurers adopt AI technologies for fraud detection, data privacy becomes a paramount concern. Ethical data handling practices are essential in preserving customer trust and complying with privacy laws. Insurers must ensure that data used for training AI algorithms is anonymized and compliant with regulations such as GDPR.
The public must feel confident that their information is handled responsibly, and AI systems should be transparent about how data is utilized. This transparency plays a crucial role in establishing trust in the technology while simultaneously defending against fraudulent practices.
Mitigating Bias in AI Models
Bias within AI models can lead to unfair treatment of certain groups or individuals, making it imperative for insurers to actively work to identify and mitigate biases. This task involves scrutinizing the data fed into algorithms and ensuring a diverse training dataset that accurately represents the entire insured population.
Implementing strategies such as regular bias audits and refining algorithms based on diverse inputs can enhance fairness in fraud detection processes, contributing to more equitable outcomes for all policyholders.
Regulatory Compliance: Navigating Legal Frameworks
The regulatory landscape surrounding AI in insurance is evolving, and staying compliant is critical for all insurers. Adhering to regulations not only fosters public trust but also safeguards against potential legal repercussions. Insurers must ensure that their AI-driven systems align with existing laws and standards while remaining adaptable to future regulatory changes.
Implementing an effective governance framework is essential to navigate these complexities, providing guidance on ethical AI practices and compliance requirements for insurers.
Conclusion
AI is transforming fraud detection within claims automation, providing a formidable tool for insurers to combat deceptive behaviors while enhancing operational efficiency. By leveraging AI’s capabilities, insurers can significantly improve accuracy in detecting potential fraud, keeping pace with evolving schemes and ensuring swift resolutions for legitimate claims. As we look to the future, the impact of AI will only continue to grow, reshaping the insurance landscape. To learn more about how Inaza can assist in implementing AI-driven solutions in claims automation, check out our related blog on how data enrichment improves underwriting accuracy. For personalized guidance, contact us today.