AI-Driven Severity Assessment: Prioritizing High-Risk Bodily Injury Claims
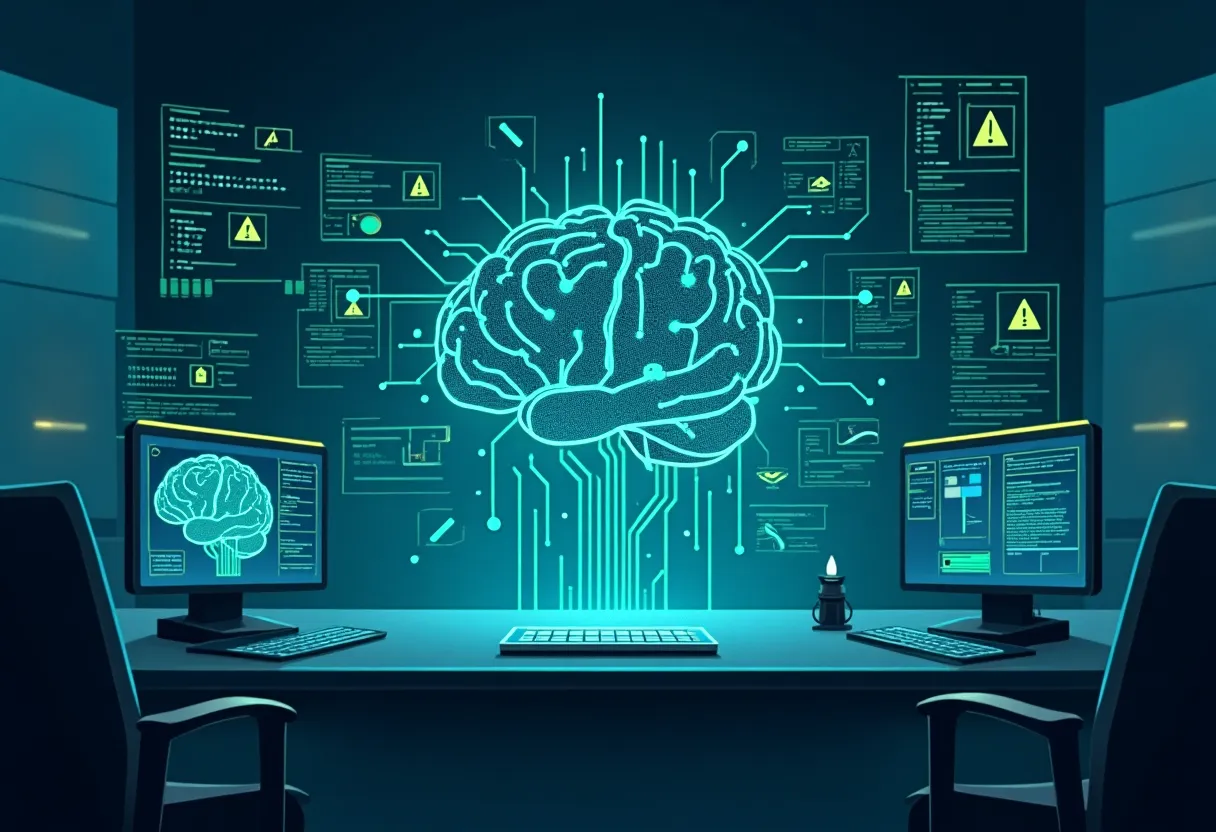
Introduction
In the insurance industry, particularly in the realm of bodily injury claims, effective severity assessment is critical. It serves as the foundation for determining the appropriate response and financial settlement associated with claims of this nature. As the complexity of these claims increases, so does the importance of leveraging innovative technologies, especially artificial intelligence (AI). The transformative impact of AI on the insurance sector is profound, as it introduces new methodologies for assessing risk and streamlining workflows.
By prioritizing high-risk claims through AI-driven severity assessments, insurers can not only expedite the process of settling claims but also ensure that the outcomes are fairer for all parties involved. This methodical approach allows for a more comprehensive understanding of potential liabilities, ultimately leading to more accurate settlements and improved operational efficiency.
What is AI-Driven Severity Assessment and Why Does It Matter?
How Does AI Function in Severity Assessment?
AI-driven severity assessment utilizes machine learning algorithms and data analytics to evaluate the factors that contribute to the severity of bodily injury claims. These AI systems analyze large datasets, encompassing historical claims, medical records, and other pertinent information, to identify patterns and predict outcomes. The mechanisms include data processing capabilities that account for a multitude of variables, such as the nature of the injury, typical recovery times, and previous case settlements.
This innovative approach enables insurers to quickly categorize claims based on their risk levels, allowing them to focus their resources where they are most needed. Furthermore, by utilizing predictive models, AI helps in estimating future claims costs, which plays a critical role in financial forecasting and risk management.
What is the Role of Predictive Injury Assessment?
Predictive injury assessment is a vital component of AI-driven severity assessments. By employing statistical methodologies and past data, insurers can develop models that forecast the potential severity and costs associated with current claims. This proactive stance allows for key decisions to be made early in the claims process, resulting in an overall reduction of claim cycle times and improved claimant satisfaction.
Moreover, predictive injury assessments make it possible to highlight outliers – claims that may present unusual risks or costs. This granular level of detail not only assists adjusters in doing their jobs more efficiently but also reduces the likelihood of expensive or contentious disputes arising later on in the process.
Why is Prioritizing High-Risk Claims Crucial for Insurers?
High-risk claims require immediate attention because they hold the potential for significant financial exposure. By prioritizing these claims, insurers can mitigate risks and avoid unforeseen losses that could arise from delayed assessments. In this regard, an efficient AI-driven severity assessment becomes paramount. It ensures that the claims that are likely to incur the greatest costs are addressed first.
This systematic approach does not merely benefit insurers. Faster resolution of high-risk claims can lead to quicker settlements for claimants, improving customer satisfaction and trust in the insurer. Ultimately, prioritizing these claims serves to create a more sustainable and responsive insurance environment.
How are AI and Medical Damage Assessment Interconnected?
What is Medical Damage AI and its Application in Insurance?
Medical damage AI refers to the application of artificial intelligence technologies to assess medical damages associated with bodily injury claims. This technology examines medical records, treatment histories, and various clinical data points to determine the extent of injury and a fair compensation amount. In essence, it provides insurers with a data-backed analysis that enhances their understanding of each claim's complexity.
The integration of medical damage AI into insurance workflows minimizes human error and enhances objectivity in evaluations, allowing adjusters to rely on accurate, data-driven insights rather than conjectures or incomplete information.
How Does AI Analyze Medical Records for Risk Assessment?
AI analyzes medical records using advanced natural language processing (NLP) techniques to extract relevant information promptly and accurately. This information can include treatment plans, doctor notes, imaging results, and even insurance claim histories. By synthesizing this data, AI systems can calculate risk factors associated with each claim, giving insurers a robust tool for assessing potential liabilities.
Additionally, by cross-referencing historical data with current claims, AI can identify potential red flags, such as chronic conditions or past claims, that may influence the current case's outcome. This ability to analyze medical records in depth reinforces the insurer's capacity for informed decision-making.
What Are the Benefits of Automated Medical Damage Assessment?
The benefits of an automated medical damage assessment are myriad. Key advantages include:
- Increased efficiency: Automated processes lead to quicker evaluations, allowing insurers to reduce claim processing times.
- Enhanced accuracy: Automated tools mitigate human errors associated with manual assessments.
- Comprehensive assessments: AI can factor in a wide array of data points, leading to more accurate liability determinations.
- Cost-effectiveness: Reducing the time and resources spent on assessments can lead to significant savings for insurers.
What Processes Are Involved in Automated Demand Prioritization?
How Does AI Streamline the Claims Processing Workflow?
AI significantly streamlines the claims processing workflow by automating routine tasks and providing sophisticated analytics tools for decision-makers. The automation of data entry, document verification, and preliminary assessments reduces the burden of manual workload on claims adjusters. Furthermore, AI-driven analytics help prioritize claims based on their potential impact and the decisiveness of required actions.
Such streamlined workflows not only improve turnaround times but can also foster a more positive interaction between insurers and their claimants. This, in turn, leads to enhanced relationships and could result in an uptick in customer loyalty.
What Techniques are Used in Automated Demand Prioritization?
Several techniques facilitate automated demand prioritization. Machine learning algorithms play a significant role in evaluating historical data to create a risk profile for new claims. This risk profiling often incorporates various factors, including the claimant’s history, the nature of the injury, and the associated treatment required.
Additionally, clustering methods can identify patterns among claims, placing them into specific categories based on similarities. By doing so, insurers can allocate their resources effectively, ensuring that high-risk claims receive the attention and expertise they warrant.
How is Data Aggregation Important in Claims Prioritization?
Data aggregation is crucial in establishing a comprehensive view of a claim’s potential severity. By combining data from multiple sources—medical records, previous claims data, accident reports, and more—insurers can form a well-rounded assessment of risk factors. This holistic view allows for more informed prioritization in the claims process.
Furthermore, centralized data repositories equipped with AI capabilities enhance the processing of real-time data, enabling insurers to quickly adapt to changing circumstances and emerging risks.
How Can High-Risk Claims Impact Loss Ratios and Profitability?
What is the Relationship Between High-Risk Claims and Financial Stability?
High-risk claims directly impact an insurer's loss ratios, which in turn affects profitability. Loss ratios, or the ratio of claims paid out to premiums earned, must be managed diligently to maintain financial health. A higher frequency of high-risk claims can lead to elevated losses, which can jeopardize not only individual insurers but also the overall stability of the insurance market.
Effective management of high-risk claims through AI-driven methodologies can mitigate these impacts by allowing insurers to make more strategic decisions regarding reserve allocations and risk assessments.
How Can AI Mitigate Risks Associated with Bodily Injury Claims?
AI addresses risks associated with bodily injury claims in several ways. By leveraging predictive analytics, insurers can identify high-risk cases early in the process. Additionally, AI helps in optimizing claims adjustments based on an extensive historical analysis, ensuring that claims settlements reflect actual liabilities.
Moreover, AI systems can detect patterns of fraudulent claims, reducing instances of unnecessary payout and protecting the insurer's bottom line. This proactive approach to risk management leads to more sustainable underwriting practices and improved financial performance.
What are the Challenges in Implementing AI in Claims Assessment?
How Do Data Privacy Regulations Influence AI Implementation?
Data privacy regulations play a significant role in shaping AI implementation within the insurance industry. Insurers must navigate a complex landscape of compliance, ensuring that consumer data is managed ethically and in accordance with applicable laws. This is especially important when employing AI tools that utilize sensitive medical and personal information.
Failure to comply with these regulations can result in severe penalties and damage to the insurer's reputation, making awareness and adherence to privacy laws paramount in AI strategies.
What Are Common Misconceptions About AI in Insurance?
Several misconceptions can hinder the effective implementation of AI in the insurance sector. One prevailing belief is that AI will replace human adjusters entirely. In reality, AI is designed to augment human capabilities, making the claims process more efficient but still reliant on human judgment and expertise for complex cases.
Another misconception is that AI systems require vast amounts of data to be effective. While high-quality data is essential, AI techniques can be surprisingly effective even with limited datasets, thanks to advancements in transfer learning and few-shot learning methodologies.
How Can Insurers Overcome Barriers to AI Adoption?
Insurers can overcome barriers to AI adoption by fostering a culture of innovation and continuous learning within their organizations. This includes investing in training and development for their workforce, ensuring that employees are adept at understanding and utilizing AI technologies.
Engagement with technology partners and stakeholders is also essential, as collaboration can facilitate smoother transitions and provide necessary technical expertise. By creating strategic alliances, insurers can effectively integrate AI into their claims processes and enhance operational capabilities.
How Should Insurers Approach the Transition to AI-Driven Solutions?
What Steps Should Be Taken for Successful Implementation?
Successful implementation of AI-driven solutions requires careful planning and strategic execution. Insurers must first identify the specific areas within claims processing that can benefit from AI technologies. This often entails conducting a thorough analysis of existing operations to pinpoint inefficiencies and potential improvement areas.
Following this, establishing clear objectives and metrics for success is essential. Insurers can address technical challenges and ensure that the integration of AI solutions aligns with their overall business goals.
How Can Training and Change Management Impact Adoption?
Training and change management are critical components of the successful adoption of AI solutions in insurance. Providing employees with the necessary skills and resources empowers them to leverage new technologies effectively. Change management processes that embrace open communication and support help ease any resistance and cultivate a more engaged workforce.
This cultural shift can be instrumental in creating an environment where innovation thrives and employees actively participate in driving progress in AI application.
What Metrics Should Be Used to Evaluate AI Effectiveness in Claims?
Evaluating the effectiveness of AI in claims processing necessitates a set of well-defined metrics. Key performance indicators (KPIs) may include claim processing times, accuracy of damage assessments, customer satisfaction scores, and reduction in fraudulent claims. Regularly monitoring these metrics enables insurers to gauge the ROI of their AI initiatives and identify opportunity areas for further enhancement.
In conclusion, the continuous refinement of AI capabilities should remain a focal point as insurers balance operational efficiency with the need for risk management. The ongoing evolution of AI presents great potential for enhancing the insurance landscape.
What is the Future of AI in Bodily Injury Claims and Severity Assessment?
How Will Machine Learning Evolve in Insurance Practices?
The future of AI in bodily injury claims is closely tied to the evolution of machine learning practices. As algorithms become more sophisticated, the accuracy of predictive models will improve, allowing for even more precise assessments and claims prioritization. These advancements will not only enhance decision-making processes but also refine risk management strategies, resulting in substantial cost savings for insurers.
What Innovations Should We Anticipate in Automated Assessment?
Anticipated innovations in automated assessment include the integration of AI with blockchain technology, significantly enhancing data security and transparency. Additionally, advancements in natural language processing will allow for more nuanced interpretations of medical records and claimant narratives, further improving assessment accuracy.
The continued convergence of AI with other data management solutions is expected to create an ecosystem where insurers can make faster, smarter decisions grounded in comprehensive, real-time data analysis.
How Will AI Shape Consumer Expectations and Experiences?
As AI continues to transform the claims process, consumer expectations will also evolve. Claimants will increasingly expect rapid responses, transparent processes, and fair settlements. Insurers that harness AI effectively will be better positioned to meet these expectations, leading to improved customer experiences and heightened brand loyalty.
Ultimately, the insurance landscape is on the brink of fundamental change driven by AI. Insurers that adapt to these advancements will benefit from enhanced operational efficiencies while delivering superior service to their clients.
Conclusion
The potential of AI-driven severity assessment in the insurance industry is nothing short of transformative. By prioritizing high-risk bodily injury claims, insurers can facilitate faster and fairer settlements, paving the way for enhanced operational efficiency and improved claimant satisfaction. As the landscape continues to evolve, embracing AI will be crucial for navigating the complexities of claims management.
As the journey towards embracing AI in insurance unfolds, it's essential for insurers to stay attuned to ongoing developments in technology and adapt accordingly. For those interested in taking the next step, explore our Email Automation solution to see how it can enhance your claims processing efforts and improve operational efficiency.