AI-Powered Signature Analysis for Detecting Forged Insurance Documents
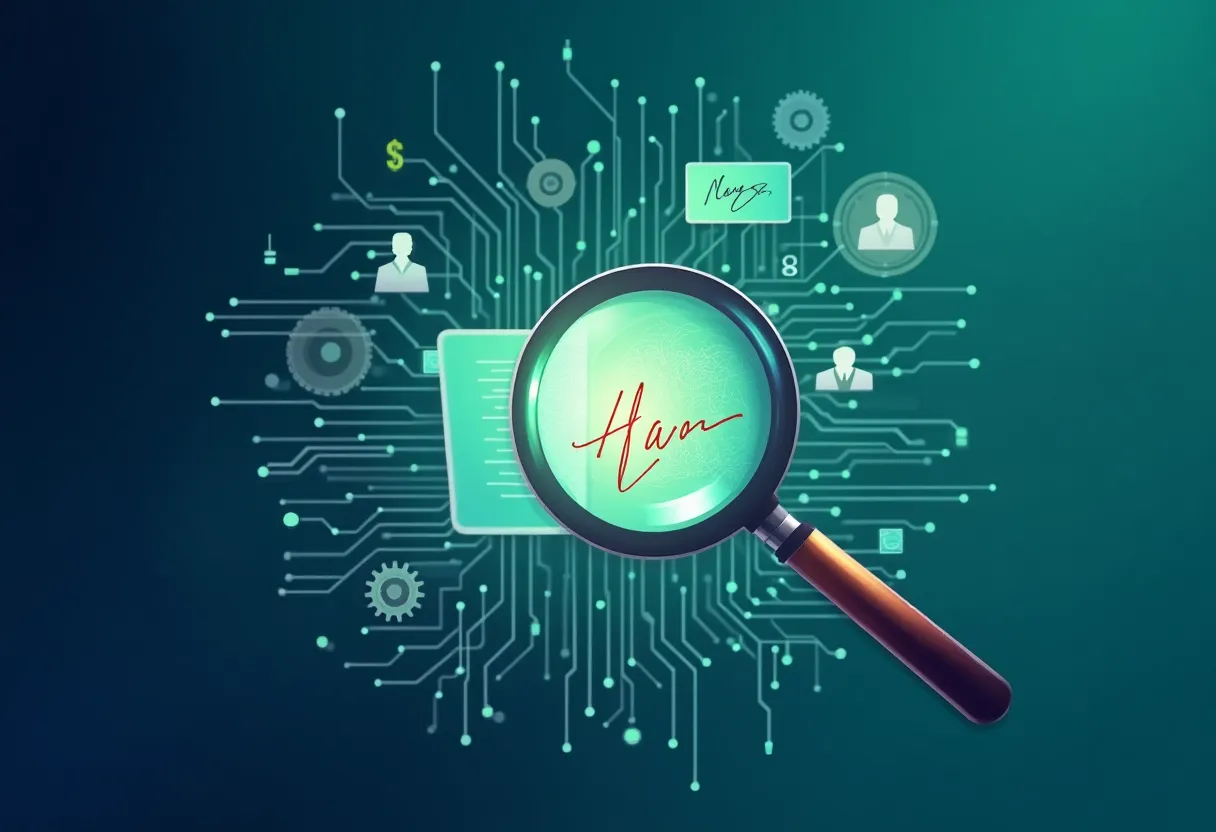
Why is Signature Analysis Important in the Insurance Sector?
Signature analysis plays a crucial role in the insurance sector by ensuring the authenticity of documents. Insurance contracts, claims forms, and various legal instruments hinge on signatures as a form of identity verification. These signatures substantiate agreements and validate information provided by policyholders. As such, their integrity is paramount; a fraudulent signature can invalidate a claim and cost insurers significant losses.
Forged signatures can have drastic implications on insurance claims. When a signature is forged, it generally leads to the approval of illegitimate claims, resulting in financial losses for insurers and increased premiums for honest policyholders. The burgeoning problem of signature fraud not only threatens financial stability but also the trustworthiness of the insurance industry as a whole. Traditional methods of detecting these forgeries, while useful, often fall short in speed and accuracy, leaving insurers vulnerable to fraudsters.
In contrast, AI-powered solutions for signature analysis provide a revolutionary alternative. Unlike conventional methods that rely on manual comparisons, AI systems can analyze massive amounts of data quickly and much more accurately. Utilizing advanced algorithms, these tools can detect inconsistencies and anomalies that the human eye simply cannot, thus significantly enhancing the efficiency and security of the document verification process.
How Does AI-Powered Signature Analysis Work?
AI-driven signature analysis is largely grounded in machine learning techniques that enable computers to learn from data. Essentially, machine learning models are trained on vast datasets containing both genuine and forged signatures. By analyzing patterns and features, these algorithms can discern the subtle differences between authentic and fraudulent signatures. Over time, as they process more examples, the accuracy of these models improves significantly, leading to more reliable verification outcomes.
Several key technologies underlie AI signature analysis, including neural networks and convolutional neural networks (CNNs). These technologies are particularly adept at image recognition tasks and can be employed to identify and categorize signature characteristics. Incorporating these technologies enhances the effectiveness of signature verification by enabling the system to learn complex patterns that signify authenticity or forgery.
Image processing also plays a pivotal role in signature recognition. By applying techniques such as edge detection, contour mapping, and feature extraction, AI systems can analyze signatures at a granular level. As a result, they can pinpoint variations in pressure, stroke order, and spatial arrangement—elements that human analysts might overlook. This multi-layered approach not only increases the accuracy of signature verification but also expedites the processing time compared to traditional methods.
What is Signature Counting and How is it Applied?
Signature counting refers to the process of quantifying signatures on documents to aid in verification efforts. It holds particular relevance in the context of insurance, where multiple signatures on claims or contracts need to be evaluated for authenticity. By applying signature counting algorithms, insurers can systematically assess whether the number of signatures meets expected counts and identify any discrepancies that may indicate forgery.
Signature counting algorithms often function by recognizing and analyzing individual signatures within a document. They extract relevant features, such as size and stroke style, to distinguish between distinct signatures. Through machine learning techniques, these algorithms can be trained to understand what constitutes a genuine signature and flag instances where the count appears abnormal, warranting further investigation.
For example, offices handling multiple claims may see a rise in incidents where an unusually high number of claims are signed by the same individual. In these cases, signature counting can play a vital role in fraud detection, ultimately preventing insurers from inadvertently processing and paying out on illegitimate claims.
How Can AI Enhance Document Security?
Document security is paramount in an industry as sensitive as insurance. AI enhances document security through advanced techniques that bolster detection rates for forged documents. These techniques involve deep learning models that analyze not only signatures but also the overall metadata and formatting of documents to determine authenticity. By looking for discrepancies in typesetting, document structure, and signature formation, AI algorithms can identify potential forgery risks.
Robust document security protocols are essential to maintaining integrity and preventing fraud. These protocols may include regular updates to verification algorithms, employee training on identifying red flags, and the integration of AI tools capable of rapid verification. Maintaining strict compliance with regulatory guidelines also helps to safeguard against fraud and ensures that insurers are protected from unwarranted exposure.
What are the Benefits of AI-Driven Compliance in Insurance?
AI-driven compliance solutions streamline the process of aligning regulatory requirements with technology solutions. Insurers must adhere to stringent guidelines that govern the verification of documents and claims. By leveraging AI, companies can automate compliance checks, ensuring that each step of the process is thoroughly examined and adheres to established standards.
Reducing risk through AI-driven compliance checks can result in lower liability for insurers and diminish the potential for penalties due to oversight. Automated checks seamlessly weave into existing workflows, minimizing the chances of human error and ensuring data integrity. This long-term investment in automated compliance processes pays off by not only improving operational efficiency but also enhancing consumer trust in the insurer's practices.
How Can Insurers Prevent Fraudulent Claims with AI?
Predictive analytics is increasingly recognized as a powerful tool in fraud prevention for insurers. By analyzing historical data, AI systems can identify patterns indicative of fraudulent behavior. These insights provide a proactive measure to spot suspicious activities before they escalate into significant financial losses.
The process of flagging and investigating suspected fraud also moves swiftly with the integration of AI in claims processing workflows. Once irregularities are detected, the AI can alert claims adjusters, providing them with detailed analyses to support further investigations. This heightened level of scrutiny not only deters potential fraudsters but assures policyholders that their claims will be assessed fairly and diligently.
What Challenges Do Insurers Face with Signature Analysis?
Despite the promise of AI in transforming signature analysis, insurers face several challenges. Recognizing the limitations of current technologies remains a priority. While AI can significantly enhance accuracy, the technology still requires human oversight, particularly in complex cases where subtle nuances in handwriting come into play. Accurately interpreting machine outputs without adequate human context can lead to misjudgments.
Moreover, insurers often encounter resistance to AI implementation from staff wary of technological shifts. This resistance can stem from concerns about job displacement or skepticism regarding the effectiveness of AI solutions. Educating employees on the benefits of AI and involving them in the implementation process are crucial steps in overcoming these hurdles.
Ensuring accurate training data for AI models is pivotal for success. For AI systems to learn effectively, they must be exposed to diverse datasets that include various handwriting styles, age groups, and cultural signatures. Failure to provide a robust training set can result in biased models that exacerbate the issues they were designed to mitigate.
What is the Future of AI in Insurance Signature Verification?
The future of AI in insurance signature verification appears bright as the technology continues to evolve. Emerging trends include the advancement of natural language processing and contextual analytics, which can further enhance the ability to assess document integrity. As these technologies mature, we can expect greater accuracy and efficiency in identifying forged documents.
The potential for further integration of AI within the insurance landscape is vast. With continuous developments, insurers can adopt more sophisticated tools capable of conducting real-time analysis, thus providing rapid decision-making support. As the industry leans into these innovations, the barrier for entry into fraudulent claims will only heighten, benefiting all stakeholders involved.
Predictions indicate that signature analysis will grow increasingly autonomous, leveraging AI to not only verify signatures but also process entire documents with minimal human intervention. Digital transformation will continue shaping the future of insurance, emphasizing the growing need for innovative and secure methods for document validation.
Conclusion
In summary, AI-powered signature analysis is pivotal in safeguarding the insurance sector against fraud and enhancing document security. By adapting to these evolving technologies, insurers can bolster their defenses against fraudulent activities, ensuring greater integrity in their processes. Embracing AI not only enhances operational efficiency but also establishes greater trust with policyholders.
For a deeper dive into how signature analysis can transform your insurance operations, explore our AI-powered signature verification solutions. Don't hesitate to contact us today to learn more about how our innovations can secure your documents and protect your business.