How AI Reduces False Positives in Fraud Detection
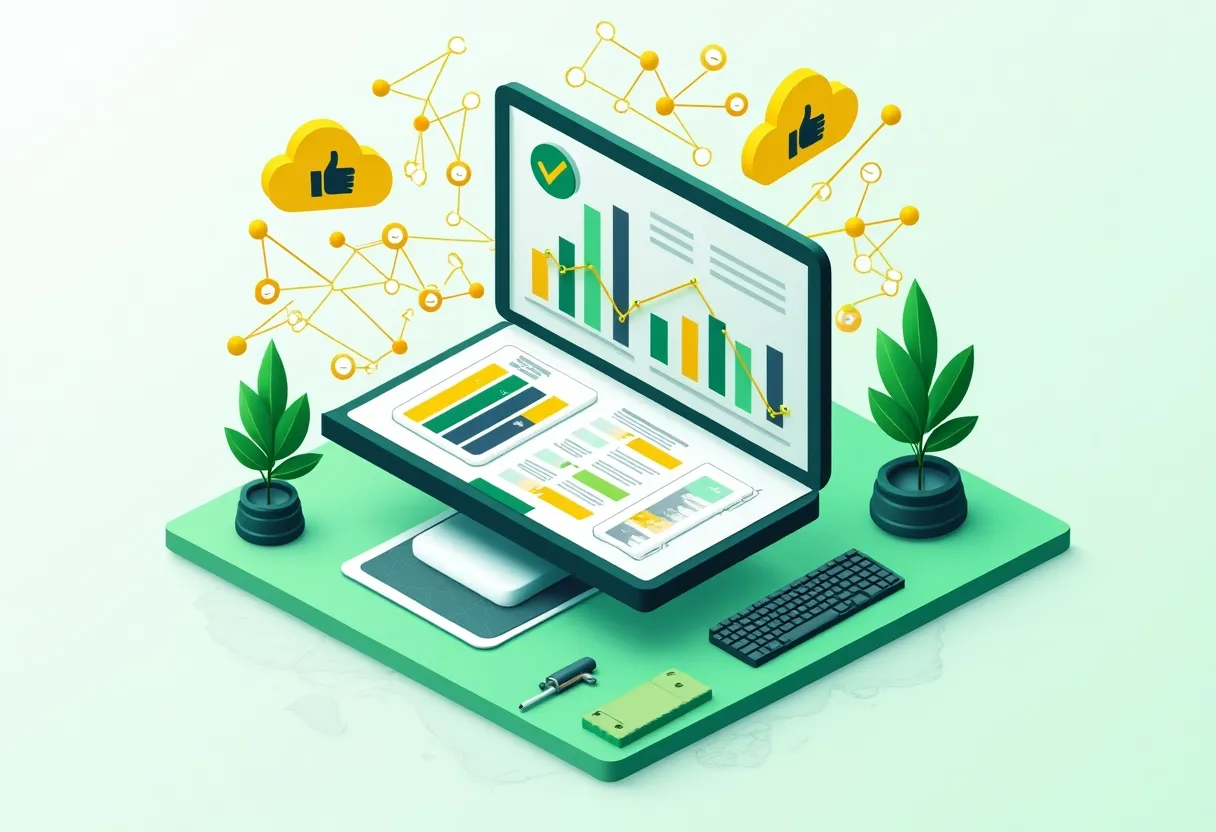
Introduction
Fraud detection in the insurance industry has become increasingly vital as fraudulent activities grow in complexity and sophistication. The financial implications of fraud can be staggering, and effective fraud detection systems are paramount in safeguarding insurers' interests. However, a significant challenge within these systems is the prevalence of false positives—situations where legitimate claims are mistakenly flagged as fraudulent. This not only results in operational inefficiencies but also dampens customer satisfaction. Fortunately, the advent of artificial intelligence (AI) presents transformative opportunities to minimize these false positives, enhancing both the accuracy and efficiency of fraud detection processes.
What Are False Positives in Insurance Fraud Detection?
Definition of False Positives
A false positive in insurance fraud detection is defined as an instance where a legitimate insurance claim is incorrectly identified as fraudulent by the detection system. This misclassification can occur due to various factors, including the complexities of human behavior and the nuances of individual claims.
The Impact of False Positives on Insurers
The impact of false positives on insurers is multifaceted and can severely disrupt operations. Each false positive results in unnecessary investigations, causing delays in claims processing and an inefficient allocation of resources. Insurers not only face additional operational costs, but they also risk damaging their relationships with legitimate customers, who may become frustrated by prolonged claims processing times and feeling wrongfully accused.
Common Causes of False Positives in Traditional Systems
Traditional systems often rely on heuristic algorithms and rule-based criteria to flag potential fraud. However, these methods can be overly simplistic and fail to account for the diverse nature of legitimate claims. Common causes of false positives include rigid thresholds for certain attributes, lack of contextual analysis, and insufficient data integration from various sources.
How Does AI Enhance Fraud Detection?
Overview of AI Technologies in Fraud Detection
AI technologies are revolutionizing fraud detection in the insurance industry through their capability to analyze vast volumes of data quickly and accurately. By leveraging sophisticated algorithms and machine learning models, AI systems can discern patterns and make more nuanced determinations about the legitimacy of claims. These advancements provide insurers with the tools needed to enhance their fraud detection capabilities significantly.
Machine Learning Algorithms and Their Role
Machine learning (ML) algorithms play a crucial role in AI-powered fraud detection systems. These algorithms learn from historical data and continuously improve their performance over time, allowing them to adapt to new fraud patterns. Unlike traditional systems, which operate on fixed criteria, ML algorithms can adjust their parameters based on emerging trends, thus increasing their accuracy in identifying legitimate claims and reducing false positives.
Comparing Traditional vs. AI-Powered Fraud Detection Systems
The contrast between traditional and AI-powered fraud detection systems is stark. Traditional methods often yield a high rate of false positives due to their reliance on fixed heuristics and rules. In contrast, AI-powered systems utilize dynamic learning processes and advanced statistical techniques, enabling them to assess a multitude of variables and determine the likelihood of fraud with greater precision. This shift not only enhances fraud detection efficacy but significantly reduces the burden on claims processing teams.
In What Ways Does AI Reduce False Positives?
Advanced Data Analysis Techniques
AI employs sophisticated data analysis techniques that facilitate the reconciliation of vast and varied datasets. These techniques enable the identification of subtle correlations that might indicate legitimate claims rather than fraudulent activities. By cross-referencing diverse data points—such as social media activity, transaction history, and previous claims—AI can construct a more comprehensive view of each claim, ultimately leading to a reduction in false positives.
Behavioral Pattern Recognition
AI-driven systems excel in recognizing behavioral patterns that distinguish between fraudulent and legitimate activities. By analyzing historical data, algorithms can identify normal behavioral trends among policyholders, making it easier to flag deviations that genuinely indicate fraud. This capability helps minimize false positives by ensuring that only genuinely suspicious claims are investigated, thus allowing legitimate claims to proceed smoothly through the processing pipeline.
Real-time Fraud Monitoring
The real-time monitoring capabilities of AI are another significant advantage in reducing false positives. Unlike traditional systems that may conduct periodic reviews, AI enables continuous surveillance of claims as they occur. This immediacy allows insurers to react promptly to suspicious activities while maintaining a clear understanding of the context surrounding each claim, further mitigating the chances of false classification.
What Benefits Does Reducing False Positives Bring to Insurers?
Decreased Operational Costs
By reducing the incidence of false positives, insurers can significantly decrease operational costs associated with investigating legitimate claims. With fewer unwarranted claims flagged for further scrutiny, resources can be reallocated to areas that improve overall operational efficiency and customer service. This efficiency translates into cost savings that can be redirected toward enhancing products and services.
Improved Customer Experience
Reducing false positives greatly enhances the customer experience. When legitimate claims are processed swiftly and without undue scrutiny, customers feel valued and supported. A positive claims experience fosters loyalty and enhances the insurer's reputation, which is crucial in an industry where trust is paramount. Happy customers are more likely to recommend their insurance provider, leading to potential new business opportunities.
Enhanced Fraud Prevention Strategies
With fewer false positives, insurers can focus on developing stronger fraud prevention strategies. AI provides insights into patterns that may indicate emerging fraud techniques, allowing insurers to stay ahead of fraudulent behavior. This proactive approach mitigates risks and enhances the overall security of the insurance ecosystem.
What Are Some Real-World Applications of AI in Fraud Detection?
Case Studies of Successful Implementations
Several insurance companies have successfully integrated AI into their fraud detection strategies, reporting significant decreases in false positives. Organizations utilizing machine learning models to evaluate claims data have found that their operational efficiency improves while minimizing interruptions to legitimate claimants. These implementations serve as blueprints for other insurers seeking to enhance their fraud detection capabilities.
Lessons Learned from AI Adoption in Insurance
The journey of AI adoption in insurance has revealed several important lessons. Ensuring data quality and diversity is fundamental for training effective machine learning models. Additionally, fostering a culture of collaboration between data scientists and insurance professionals allows for more tailored solutions that address specific industry challenges. Educating staff on AI's roles and outcomes also fosters a smooth transition and enhances user acceptance of new technologies.
Future Innovations on the Horizon
The future of AI in fraud detection holds exciting innovations on the horizon. As algorithms evolve, we can anticipate even more sophisticated predictive capabilities. Future technologies may integrate advanced natural language processing and image recognition, allowing for a multifaceted approach to data interpretation that enhances the decision-making process in fraud assessment.
How Can Insurers Implement AI Solutions to Combat Fraud?
Steps for Integrating AI into Fraud Detection Processes
Integrating AI into existing fraud detection processes begins with understanding specific business needs and identifying suitable data sources. Insurers should assess the current technology stack and explore AI solutions that can enhance existing systems. Developing strategic partnerships with AI technology providers is critical, as their expertise can guide deployment while aligning with the insurer's operational goals.
Considerations for Choosing an AI Provider
When selecting an AI provider, insurers must consider factors such as the provider's industry experience, technology scalability, and support services. A robust provider should offer transparent processes and demonstrate a commitment to integrating feedback and knowledge from the insurance landscape into their solutions. Collaborative partnerships foster innovation and ensure that the adopted technology continuously meets evolving fraud detection needs.
Building a Culture of Continuous Improvement with AI
A culture of continuous improvement is essential for harnessing AI's full potential in fraud detection. Organizations should encourage open dialogue about performance metrics and fraud trends, creating an environment that fosters learning and growth. Providing ongoing training ensures that staff members are equipped with the latest knowledge on AI technologies and methodologies, ultimately leading to more effective fraud detection strategies.
Conclusion
In conclusion, reducing false positives through AI technologies dramatically enhances the effectiveness of fraud detection in insurance. By utilizing advanced data analysis techniques, behavioral pattern recognition, and real-time monitoring, insurers can navigate the complexities of fraud detection while prioritizing their relationships with legitimate policyholders. The future of AI in fraud detection appears bright, paving the way for more efficient operations and a stronger defense against fraudulent activity. For further insight into automation in claims processing, you may find our related blog on how predictive models enhance automated claims processing informative. If you're ready to explore how AI can transform your fraud detection strategies, contact us today.