How Predictive Analytics Enhances Bodily Injury Claims Processing
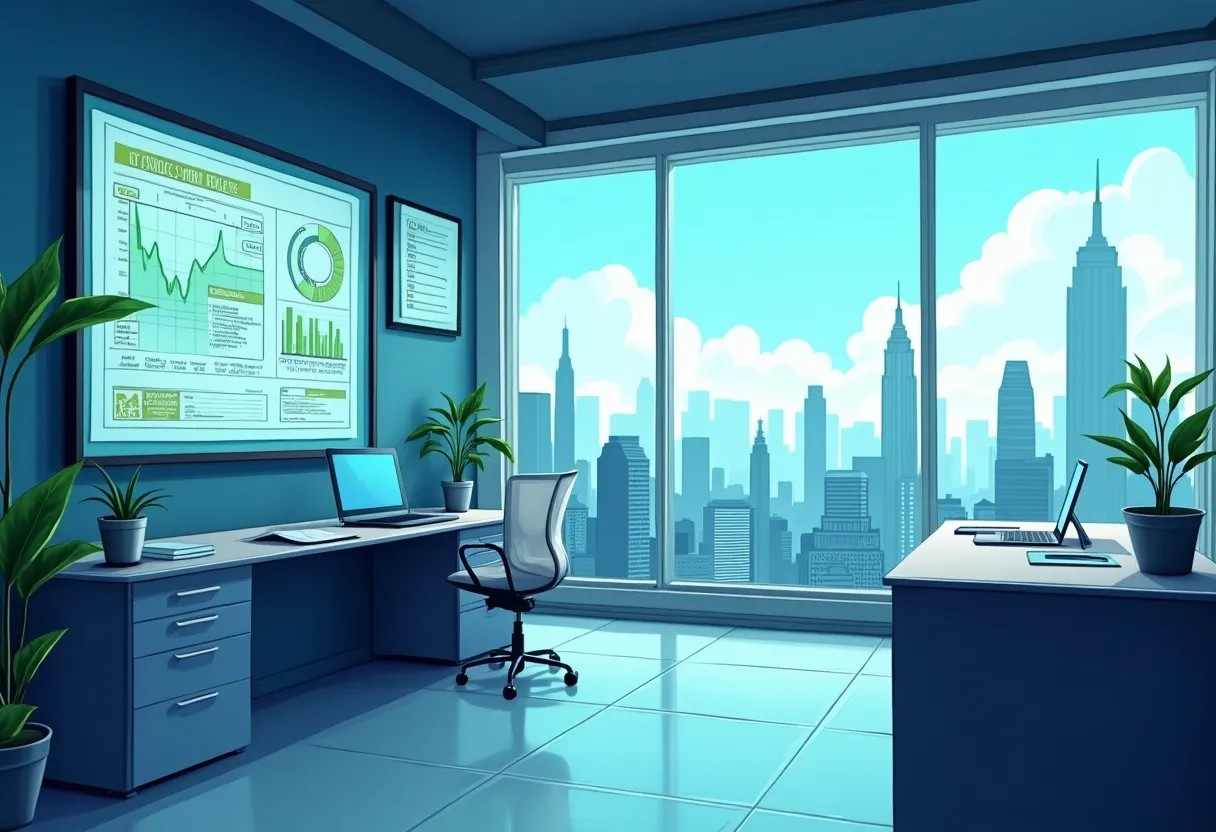
What is Predictive Analytics in Insurance?
Predictive analytics in insurance refers to the utilization of historical data, statistical algorithms, and machine learning techniques to foresee future outcomes. By analyzing patterns from previous incidents and claims, insurers can predict costs, risks, and the likelihood of certain events occurring, which ultimately allows for more informed decision-making processes.
Defining Predictive Analytics
At its essence, predictive analytics integrates multiple disciplines, such as statistics, data mining, and machine learning, to extract relevant insights from data. In the context of insurance, it serves as a powerful tool that aids insurers in identifying risk levels associated with policies, enhancing underwriting processes, and optimizing claims management strategies. This use of analytics has evolved considerably over the years, adapting to the rapidly changing technological landscape.
Historical Context of Predictive Analytics in Claims Processing
The journey of predictive analytics in the insurance sector has its roots in credit scoring and risk assessment, which began in the mid-20th century. Over the decades, advancements in technology and computing power have enabled insurers to leverage vast quantities of data to enhance their operations. By the early 2000s, predictive modeling became mainstream, empowering insurers to analyze claims more efficiently, thereby improving operational outcomes.
Key Components and Techniques Used in Predictive Analytics
There are several key components that make up predictive analytics in insurance. First, data collection is essential, gathering historical data from various sources, including customer interactions, past claims, and market trends. Second, statistical techniques such as regression analysis and decision trees are employed to identify relationships and patterns. Finally, machine learning algorithms continuously refine results by learning from new data, making predictions even more accurate over time. These techniques, combined with advanced categorization and clustering methods, enhance the insurance landscape significantly.
How Does Predictive Analytics Improve Claims Efficiency?
Integrating predictive analytics within claims processing enhances efficiency in several key areas, enabling insurers to respond to claims promptly while reducing operational costs.
Streamlining Data Collection
One of the primary means by which predictive analytics enhances claims efficiency is through the streamlining of data collection. By automating data entry and management processes, insurers can rapidly gather necessary information from disparate sources, such as databases, internet platforms, and customer input. This simplification reduces human error while allowing claims handlers to focus on decision-making and customer engagement, rather than administrative tasks.
Automated Assessment of Claims
Predictive analytics also facilitates the automated assessment of claims, which minimizes delays and speeds up resolution times. With the assistance of algorithms that evaluate the severity of a claim based on historical data and contextual factors, insurers can prioritize high-risk cases or those requiring further investigation. By automating routine assessments, businesses can reallocate resources to more complex claims and enhance the quality of service offered to policyholders.
Reducing Time Frames for Claim Resolution
Time is of the essence in claims management, and predictive analytics plays a crucial role in shortening resolution timelines. Fast and accurate estimations can lead to quicker claims settlements, thus improving customer satisfaction. By using predictive models to gauge possible outcomes, insurers can focus their efforts on resolving claims with the highest accuracy, ensuring both expediency and clarity in the process.
What Role Does Predictive Analytics Play in Fraud Detection?
Insurers face significant challenges emanating from fraudulent claims, which can undermine profitability and threaten the core of their operations. Predictive analytics serves as a formidable line of defense in combating these issues.
Identifying Red Flags in Claims
One of the salient advantages of predictive analytics is its ability to help insurers identify red flags in claims submissions. By analyzing patterns that traditionally correlate with fraudulent activities, insurers can proactively flag suspicious claims for further investigation. This not only mitigates potential losses for the insurer but also discourages individuals from attempting fraudulent claims in the first place.
Predictive Models Used for Fraud Detection
Specific predictive models leverage historical data to create profiles of potential fraud. Techniques such as neural networks, logistic regression, and anomaly detection can reveal discrepancies between reported claims and expected outcomes based on statistical modeling. Through continuous learning from new cases, these models refine their accuracy and effectiveness over time, enhancing the insurer's ability to recognize fraudulent attempts.
Case Studies of Successful Fraud Prevention
Numerous insurers are already capitalizing on predictive analytics to thwart fraudulent claims. For example, the implementation of third-party data sources combined with historical claims data has proven effective in identifying patterns indicative of fraud. As predictive models improve, insurers have reported significant enhancements in fraud detection rates, resulting in decreased losses and improved overall efficiency.
How Can Predictive Analytics Enhance Claims Decision-Making?
Effective decision-making in claims processing relies on accurate data and insights, which predictive analytics is uniquely equipped to provide.
Data-Driven Insights for Better Outcomes
Predictive analytics empowers claims adjusters with data-driven insights that lead to better outcomes and informed decision-making. By presenting refined data visualizations and comprehensive reports, adjusters can navigate complex cases with confidence, ensuring they make decisions based on accurate assessments rather than intuition.
Incorporating Machine Learning into Claims Assessment
Moreover, the integration of machine learning into predictive analytics enables insurers to optimize their claims assessment processes. Machine learning algorithms can identify trends and predict outcomes specific to different types of claims. As a result, insurers can react more effectively to emerging trends, thereby enhancing their adaptability in a constantly evolving insurance landscape.
Real-World Applications and Their Impact
These data-driven insights translate directly into improved operational effectiveness. For instance, expedited processes for claims approval have been documented through real-world case studies where predictive analytics significantly reduced the average time taken for claims resolution, leading to increased customer satisfaction and retention rates.
What Are the Benefits of Using Predictive Analytics for Bodily Injury Claims?
The integration of predictive analytics in bodily injury claims processing carries a myriad of benefits that can contribute to both operational efficiency and superior customer experiences.
Improved Accuracy in Claim Estimates
Firstly, predictive analytics enhances the accuracy of claim estimates by utilizing a robust dataset to analyze historical outcomes comprehensively. This level of precision benefits the insurer, as they can allocate reserves more accurately and decrease the occurrence of disputes with policyholders, which can lead to smoother claim settling processes.
Enhanced Customer Experience
Furthermore, improved claims efficiency fosters a better overall customer experience. By expediting the claims process and providing timely updates, insurers can significantly enhance customer satisfaction. In an increasingly competitive marketplace, satisfied customers are likely to share their positive experiences, leading to customer retention and new business opportunities.
Reduction of Overall Claim Costs
Lastly, the automation of claims processes and the principles of predictive analytics lead to overall reductions in claim costs. With better risk assessments and more efficient resource allocation, insurers can significantly decrease the operational costs associated with claims management, ultimately benefitting their bottom line.
How Do Predictive Analytics Tools Integrate with Existing Claims Processing Systems?
The seamless integration of predictive analytics tools is crucial for their effective use within existing claims processing systems.
Compatibility with Current Infrastructure
Many predictive analytics solutions are designed with compatibility in mind, allowing them to work alongside legacy systems. This facilitates a smoother transition for organizations seeking to enhance their current capabilities without overhauling their entire infrastructure. By employing APIs and integration frameworks, insurers can implement predictive tools with minimal disruption to ongoing processes.
Customization and Scalability of Predictive Tools
Additionally, scalability is a significant advantage as insurers can tailor predictive analytics applications to meet their specific needs. As an organization grows or faces new challenges, customizable predictive tools make it possible to scale operations effectively while maintaining optimal performance.
Case Studies of Successful Integrations
Insurers that have successfully integrated predictive analytics tools report improvements in operational efficiency and enhanced decision-making capabilities. Organizations have shared their success stories, noting smoother claims processes and faster response times, further underscoring the importance of effective integration.
What Future Trends Should We Anticipate in Predictive Analytics and Claims Automation?
The future of predictive analytics in insurance is bright, with numerous trends expected to emerge in the coming years.
Evolving Technologies in Predictive Analytics
As technology continues to advance, we can expect predictive analytics tools to become even more sophisticated. With the introduction of cloud computing, big data, and enhanced data visualization techniques, insurers can gain deeper insights and foster intuitive interactions with their analytics systems.
The Growing Role of AI and Machine Learning
The integration of artificial intelligence and machine learning will further bolster the power of predictive analytics, allowing for unprecedented levels of customization and a more profound understanding of data. These technologies will empower insurers to make more rapid and precise decisions, ultimately enhancing claims processing efficiency.
Predictions for the Future Landscape of Claims Processing
In the coming years, the insurance landscape will likely witness an increased emphasis on automation and the rising significance of predictive analytics in claims processing. A proactive approach that leverages these technological advancements will lead to enhanced operational efficiencies and an elevated level of customer satisfaction.
Conclusion
In summary, predictive analytics is revolutionizing the insurance industry's approach to handling bodily injury claims by enhancing efficiency, improving fraud detection, and streamlining decision-making processes. By embracing these technological advancements, insurers gain valuable insights that can lead to better outcomes and reduced operational costs. As the insurance landscape evolves, incorporating predictive analytics tools will be crucial for entities aiming to maintain their competitive edge.
For further insights into streamlining operations, you may want to read about reducing administrative costs with straight-through processing. If you’re interested in revolutionizing your claims process, contact us today.