How to Balance Fraud Detection and Prevention Strategies
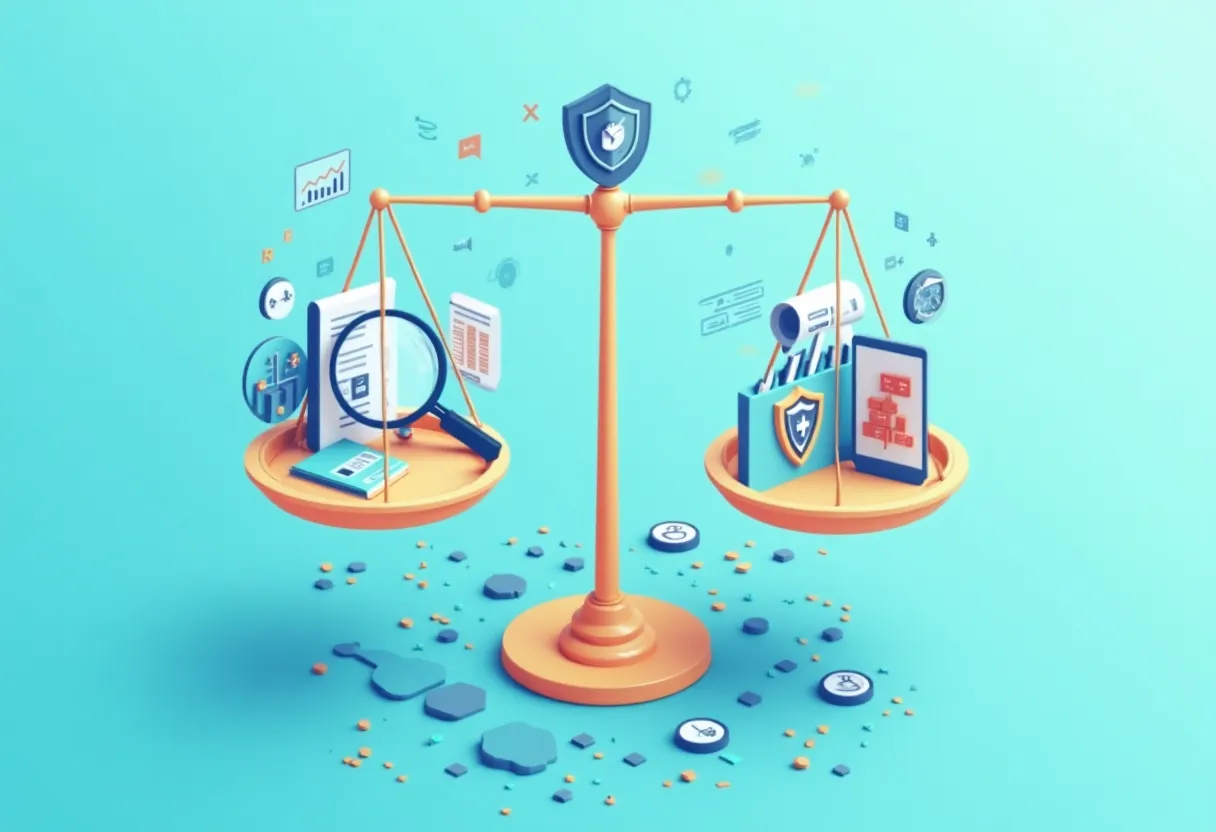
Introduction
In recent years, the insurance landscape has been increasingly challenged by sophisticated fraud schemes that evolve alongside advancements in technology. This complexity makes it imperative for insurers to strike a harmonious balance between fraud detection and prevention. By doing so, they can enhance operational efficiency, minimize losses, and ultimately improve customer satisfaction. The integration of AI and automation into these processes has become a game-changer, allowing insurers to not only detect fraudulent activities in real-time but also implement preemptive measures that mitigate risks before they escalate.
What Is the Difference Between Fraud Detection and Fraud Prevention in Insurance?
How Do We Define Fraud Detection?
Fraud detection refers to the methods and processes used to identify potentially fraudulent claims after they have been submitted. This includes real-time monitoring and assessments based on predefined criteria and patterns indicative of fraud. For instance, if a claim shows unusual patterns, such as multiple claims for similar damages by the same individual, fraud detection systems can flag these anomalies for further investigation. Examples also include the use of machine learning algorithms that analyze vast datasets to uncover hidden patterns associated with fraudulent behavior.
The importance of real-time monitoring cannot be overstated as it allows for immediate action, helping insurers minimize payouts on fraudulent claims. Advanced data analytics and predictive models are particularly valuable in this regard, enabling insurance companies to be proactive rather than reactive in their response to fraud.
How Does Fraud Prevention Differ from Detection?
Fraud prevention involves strategies implemented upfront to reduce the possibility of fraudulent claims being submitted in the first place. These strategies can range from rigorous customer verification processes during onboarding to regular training programs that heighten awareness of fraud among employees. Common fraud prevention techniques include identity verification software, robust data collection practices, and developing a culture of vigilance within the organization.
In essence, while fraud detection focuses on identifying fraudulent behavior after it has occurred, fraud prevention strives to eliminate opportunities for such behavior before it materializes. Both play critical roles but require different approaches and methodologies to be effective.
Why Is Balancing Fraud Detection and Prevention Crucial for Insurers?
What Are the Risks of Relying Too Heavily on Detection?
Relying excessively on fraud detection can lead insurers to adopt a narrow focus. One potential pitfall is that it may foster a reactive mindset, where the primary goal is to catch fraud after it occurs rather than preventing it upfront. This approach can result in elevated operational costs and inefficient resource allocation, as insurer teams become overwhelmed with the volume of fraudulent claims they are managing.
Additionally, the consequences of an unbalanced approach can be severe. For instance, insurers may find themselves spending disproportionate amounts of time on investigations while facing customer backlash due to prolonged claim processes. High-profile cases where an insurer failed to manage fraud effectively serve as cautionary tales, emphasizing the importance of a balanced approach to protect both the company’s bottom line and its reputation.
How Does Proper Balance Lead to Improved Customer Experience?
Striking the right balance between fraud detection and prevention is not just about minimizing losses; it also significantly affects customer experience. An effective balance results in faster claims processing times, minimizing delays that can frustrate policyholders. Customers are often more satisfied when they perceive the claims process as smooth and transparent.
Furthermore, when customers recognize that the insurer actively works to prevent fraud—thereby indirectly enhancing their own security—they tend to develop greater trust and loyalty towards the provider. This trust can translate into customer retention and attract new clients, thus benefiting the overall growth of the organization.
What Strategies Can Insurers Implement to Achieve Balance?
How Can AI and Automation Support Both Fraud Detection and Prevention?
AI technologies serve as a cornerstone for strategies aimed at achieving balance in fraud management. By leveraging machine learning algorithms, insurers can analyze historical data to identify emerging fraud patterns, thereby reinforcing prevention efforts. For instance, AI can aid in establishing risk profiles for policyholders based on their driving behavior, claims history, and other variables, thus enabling preventive actions tailored to specific risk poses.
Tools such as Inaza’s AI-driven fraud detection solutions exemplify how technology can streamline both fraud detection and prevention. Systems like Claims Image Recognition and FNOL automation not only help detect fraudulent submissions but also enhance customer interaction by expediting the claims process.
What Data Should Insurers Focus On for Effective Fraud Management?
Focusing on the right data types is crucial for effective fraud management. Insurers should prioritize data sources that can provide insights into customer behavior, transaction history, and claims patterns. This includes using data enrichment services to access additional information that can elucidate risk factors and behaviors indicative of fraud.
However, ethical considerations regarding consumer data handling must never be overlooked. Insurers need to ensure compliance with data protection regulations and maintain consumer trust by being transparent about how data is gathered and utilized.
How Important Is Employee Training and Awareness?
Ongoing employee training on indicators of fraud is vital for any effective fraud management strategy. Ensuring that staff are well-informed about the latest fraud trends and prevention strategies fosters a culture of vigilance and responsibility. Organizations should conduct regular training sessions and awareness campaigns to equip employees with the knowledge necessary to recognize signs of fraud in their daily operations.
To further enhance awareness, incorporating real-life case studies during training can help employees understand the practical implications of fraud and the importance of their contributions to prevention efforts.
What Role Does Claims Processing Automation Play in Balancing Efforts?
How Does Automation Improve Claims Processing Efficiency?
Claims processing automation significantly enhances efficiency by enabling straight-through processing—where claims are evaluated and completed with minimal manual intervention. This streamlining allows for automated checks and balances that flag potential fraud or inconsistencies in submissions quickly. Not only does this improve operational speed, but it also allows claims adjusters to focus on more complex cases requiring human judgment.
By utilizing tools that integrate automated decision-making, insurers can also reduce instances of human error in claims processing, further tightening fraud controls.
What Are the Limitations of Automation in Fraud Management?
Despite its many benefits, automation does have limitations. Automated systems can sometimes flag legitimate claims as fraudulent, leading to delays in legitimate payouts and potential customer dissatisfaction. Thus, while automation can enhance efficiency, it should not completely replace human oversight.
To mitigate these limitations, insurers can leverage a hybrid approach where automation handles straightforward tasks but human experts make final decisions on contentious or complex claims. This balance ensures efficient processing without compromising accuracy.
How Can Insurers Measure the Effectiveness of Their Strategies?
What Key Performance Indicators (KPIs) Should Be Used?
Measuring the effectiveness of fraud detection and prevention strategies relies on several key performance indicators. Significant metrics include the rate of fraudulent claims identified, the time taken to process claims, and the percentage of claims denied due to fraud. Additionally, tracking savings attributed to fraud prevention efforts can provide a clear picture of the financial impact of these strategies.
Establishing benchmarks for these KPIs allows insurers to regularly assess their performance and identify areas needing improvement, thus driving continuous enhancement of their fraud management strategies.
How Can Insurers Continuously Improve Their Approach?
Regular evaluation and adaptation of fraud management strategies are essential for remaining competitive in the industry. Insurers should conduct periodic reviews to assess the effectiveness of their practices against the evolving landscape of insurance fraud. Participating in industry forums and benchmarking against peers can provide valuable insights into best practices and innovative solutions.
By embracing a culture of continuous improvement and being willing to adapt methodologies based on performance metrics and industry trends, insurers can better position themselves against fraud while maintaining customer trust and satisfaction.
Conclusion
In conclusion, balancing fraud detection and prevention strategies is vital for insurers aiming to enhance efficiency while controlling potential losses. Through the integration of AI, automation, and a strong focus on employee training and data management, insurers can create a robust framework for fraud management that protects their interests while improving the customer experience. Furthermore, continuous evaluation and adaptation of strategies will ensure they remain effective in an ever-changing landscape. For further insights into how advanced technology can enhance your insurance operations, explore our previous blog on how an auto insurance PaaS can help you reach new markets.
Contact us today to learn how Inaza can empower your organization to implement effective fraud detection and prevention strategies that adapt to the evolving challenges of the insurance industry.