Improving Accuracy in Fraud Detection Systems with AI
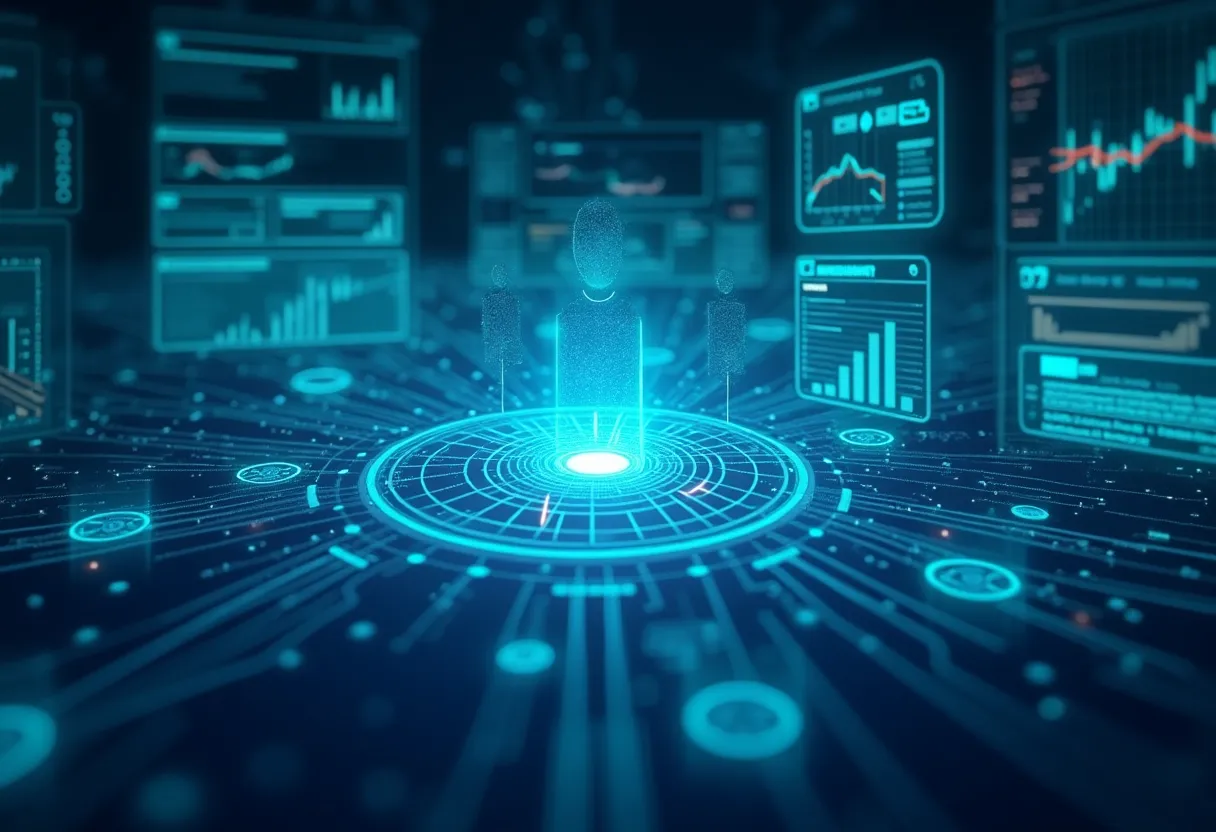
Introduction
Fraud detection stands as a cornerstone of integrity within the insurance industry, where billions of dollars are lost annually due to fraudulent claims. The pressing need for insurance companies to safeguard their operations and financial health drives the continuous improvement of fraud detection systems. However, these systems often grapple with accuracy issues, leading to a significant number of false positives—instances where legitimate claims are wrongly flagged as fraudulent. Navigating these challenges requires an innovative approach, and that’s where Artificial Intelligence (AI) emerges as a game changer.
AI offers unprecedented capabilities in enhancing the precision of fraud detection systems by improving data analysis, pattern recognition, and automated decision-making processes. This article explores the intricate role of AI in refining fraud detection accuracy while transitioning to a deeper understanding of false positives and their implications.
How Does AI Improve Fraud Detection Systems?
What are Fraud Detection Systems and their Role in Insurance?
Fraud detection systems are tools designed to identify and mitigate fraudulent activities within insurance claims. They analyze large datasets—such as claims history, customer interactions, and transactional data—to pinpoint unusual patterns that may indicate fraudulent behavior. In an industry where trust is paramount, these systems play a vital role in ensuring that resources are allocated efficiently and genuinely, while also protecting honest policyholders from the repercussions of fraudulent actions.
These systems range from rule-based structures to advanced analytics platforms that utilize machine learning. Their primary objective is to flag suspicious claims for further investigation, thereby reducing potential losses and improving operational efficiency. Nevertheless, their effectiveness is heavily influenced by the underlying methodologies employed and the quality of the data being processed.
Why Traditional Methods Fall Short in Detecting Fraud?
Traditional fraud detection methods often rely on predefined rules and heuristics to uncover potential fraudulent claims. While these approaches can be effective in certain scenarios, they have inherent limitations that can lead to inefficiencies:
- Over-reliance on historical data can miss emerging fraud schemes.
- Rigid rule-based systems often generate numerous false positives, creating a heavy burden for claims adjusters.
- Limited adaptability to evolving fraud tactics can lead to slower response times and potentially increased losses.
As fraud tactics become more sophisticated, so too must the mechanisms for detecting them. Traditional methods fall short due to their inability to learn from new data and adjust their algorithms accordingly, necessitating a shift toward AI-driven solutions.
What is the AI Advantage in Fraud Detection?
The integration of AI transforms fraud detection from a reactive to a proactive model. AI enhances these systems by providing the following advantages:
- Improved data analysis capability through advanced algorithms that can discern intricate patterns across vast datasets.
- Adaptive learning mechanisms that continuously refine detection approaches based on new data inputs and trends.
- Increased speed and efficiency in processing claims, thereby reducing turnaround times and enhancing customer service.
AI leverages machine learning, natural language processing, and predictive analytics to improve the overall accuracy of fraud detection. With the capacity to analyze thousands of data points and recognize subtle anomalies, AI systems can differentiate between legitimate and fraudulent claims more effectively than their traditional counterparts.
What are False Positives, and Why Are They a Concern?
How Do False Positives Occur in Fraud Detection?
False positives occur when legitimate claims are incorrectly classified as fraudulent due to misinterpretations or inaccuracies in the detection process. This typically arises from overly stringent rules or algorithms that fail to account for the nuances of specific claims. For instance, a genuine claim might be flagged if it falls outside typical patterns or if it possesses certain attributes that resemble known fraudulent cases.
These erroneous classifications can lead to negative experiences for policyholders and can severely hinder operational efficiency. Insurers face the dual burden of reputational damage and increased operational costs as they sort through genuine claims that have been incorrectly flagged for review.
What Impact Do False Positives Have on Insurers?
The implications of false positives extend beyond immediate financial losses. For insurers, they result in:
- Resource Drain: Increased time and effort is required to investigate false alarms, diverting resources from genuine claims.
- Customer Dissatisfaction: Policyholders may become frustrated if they face delays in claim resolutions due to their claims being misclassified.
- Financial Consequences: Operational inefficiencies stemming from the need for further investigation can accumulate significant costs over time.
Ultimately, the trust between insurers and their customers may erode as legitimate claims face unnecessary scrutiny.
What Are the Costs of Inaccurate Fraud Detection?
Inaccuracies in fraud detection translate into tangible costs for insurers, both immediate and long-term. These costs manifest in various forms:
- Loss of revenue from undetected fraudulent claims due to ineffective detection methods.
- Increased operational expenses, as teams need to chase down false positives.
- Potential legal and regulatory repercussions can emerge if accusations of fraud are unwarranted.
By employing AI-driven systems, insurers can markedly reduce these costs through improved accuracy and efficiency in their fraud detection efforts.
How Can AI Help Reduce False Positives in Fraud Detection?
What Algorithms are Effective in Identifying Fraud?
AI-powered fraud detection utilizes a variety of algorithms that enhance identification capabilities. Some of the most effective algorithms include:
- Decision Trees: These models visualize decisions in tree-like structures, delineating pathways for different outcomes based on various attributes.
- Neural Networks: Emulating human brain function, these models excel at uncovering patterns in complex data sets.
- Support Vector Machines (SVM): This classification method identifies hyperplanes that separate different classes, proving valuable in distinguishing between fraudulent and legitimate claims.
Each of these algorithms plays a critical role in ensuring a higher accuracy rate in identifying fraud while mitigating the risk of false positives.
How Does Machine Learning Improve Fraud Predictions?
Machine Learning (ML) stands at the forefront of improving fraud detection accuracy. By training algorithms on large datasets, ML can identify complex patterns that may evade traditional detection systems. As ML models process new data, they learn and evolve, allowing them to:
- Adapt to evolving fraud tactics, leading to quicker identification of new schemes.
- Enhance pattern recognition capabilities, thereby improving accuracy in predictions.
- Minimize false positives by refining criteria based on actual outcomes.
As a result, ML contributes significantly to the overall effectiveness of AI-driven fraud detection strategies.
What is the Role of Data Quality in AI-driven Systems?
The effectiveness of any AI model is intrinsically linked to the quality of data fed into it. High-quality data ensures that the algorithms are trained effectively, leading to more accurate results. Factors influencing data quality include:
- Completeness: Incomplete datasets can lead to skewed results and inadequate learning.
- Accuracy: Errors or discrepancies within the data can generate misleading outputs.
- Timeliness: Up-to-date information is essential for adapting to current fraud trends.
Insurers adopting AI-driven systems must prioritize accessing and managing high-quality data to enhance the accuracy of their fraud detection efforts.
What are the Real-World Applications of AI in Detecting Fraud?
What Are Some Case Studies of AI in Action?
Various insurers have successfully integrated AI into their fraud detection strategies, yielding promising results. For instance, companies employing machine learning models have reported substantial reductions in fraud-related losses. These applications illustrate how AI can streamline processes, quickly flagging suspicious claims for detailed analysis while allowing legitimate claims to move through the system without undue delay.
Another example includes insurers leveraging natural language processing to analyze customer communications, enabling them to detect inconsistencies or unusual phrases that may indicate fraudulent intent. These innovative applications exemplify the comprehensive capabilities AI brings to fraud detection.
How Do Insurers Implement AI for Better Accuracy?
Insurers implement AI solutions through a phased strategy comprising several steps:
- Data Collection: This initial phase focuses on gathering relevant historical data, including previous claims, customer profiles, and market trends.
- Model Development: Data scientists develop and train AI models, employing historical data to recognize patterns and anomalies.
- Integration: The models are integrated into existing claims management systems, enhancing real-time analytical capabilities.
- Evaluating Performance: Continuous monitoring and evaluation of model performance ensure ongoing accuracy.
Such structured implementation ensures seamless integration and fosters a culture of sustainability in the use of AI for fraud detection.
What Tools and Technologies Support AI Integration?
A wide range of tools and technologies facilitate the integration of AI into fraud detection systems. Data analytics platforms, machine learning frameworks, and cloud-based solutions combine to create an environment conducive to advanced fraud detection techniques. Industry-standard software, such as Python, R, and various neural network frameworks, provides insurers with the necessary resources to construct and deploy effective AI models.
Equally important is the role of data visualization tools in interpreting the results generated by AI models, allowing insurers to better understand risk patterns and refine their strategies accordingly.
What Are the Challenges of Implementing AI in Fraud Detection?
What Transition Challenges Do Insurers Face?
Transitioning to AI-driven fraud detection systems can pose various challenges for insurers. Resistance to change is a common obstacle, as stakeholders may be hesitant to trust new methodologies over established ones. Additionally, there could be a skills gap where current staff may lack the requisite knowledge or training to effectively operate new AI systems.
Furthermore, integrating AI into legacy systems can lead to technical difficulties, requiring time, resources, and often significant investment. Insurers need to confront these challenges with strategic planning and stakeholder buy-in.
How Can Data Privacy and Security Issues Be Managed?
Data privacy is paramount when implementing AI systems, as insurers must handle sensitive customer information. Mitigating these risks involves adhering to stringent data protection regulations, such as GDPR and CCPA, along with implementing robust encryption and access control mechanisms. Training employees on data privacy best practices and employing audit trails can bolster security measures while assuring customers that their data is handled responsibly.
What Skills Are Necessary for a Successful AI Implementation?
Success in implementing AI solutions for fraud detection requires a multidisciplinary team with various skills, including:
- Data Science Expertise: Professionals must possess a deep understanding of machine learning algorithms and analytical frameworks.
- Domain Knowledge: Familiarity with insurance processes and industry-specific fraud patterns is essential for effective model development.
- IT and Security Proficiency: IT specialists must ensure that the systems are securely integrated and compliant with regulatory standards.
Recruiting and training the right talent is crucial for reaping the benefits of AI-driven fraud detection systems.
What are the Future Trends in AI and Fraud Detection Systems?
How Will AI Continue to Evolve in the Insurance Space?
The landscape of AI and fraud detection will continue to evolve, marked by advancements in technology and a growing focus on customer-centric solutions. As the insurance sector increasingly adopts AI-driven methodologies, the pressure raises for ongoing innovation to keep pace with evolving fraud tactics.
Moreover, the integration of other technologies, such as blockchain, will enhance data integrity and security, further bolstering the effectiveness of AI systems in fraud detection.
What Innovations are on the Horizon for Fraud Detection?
Future innovations in fraud detection will likely include further advancements in machine learning algorithms that can incorporate unstructured data and enhance predictive capabilities. Adaptive learning systems that refine themselves based on continuous data flow will significantly reduce false positives, creating smoother workflows for insurers.
Additionally, the exploration of artificial intelligence’s role in virtual assistants for real-time claim processing may streamline communication with users and clients, reducing fraudulent engagement opportunities.
How Do We Prepare for the Next Wave of AI in Insurance?
Preparing for the next wave of AI implementation necessitates a proactive approach. Insurers should establish partnerships with technology providers and invest in ongoing training programs to ensure teams are proficient with the latest technologies. Implementing pilot programs for testing new AI solutions allows for manufacturing insights and debugging potential issues before full-scale deployment.
Finally, maintaining a flexible mindset geared toward innovation will position insurers favorably as they navigate the highly dynamic landscape of AI-enhanced insurance fraud detection.
Conclusion
In conclusion, the deployment of AI in fraud detection systems significantly reduces false positives, enhancing accuracy while safeguarding against fraudulent activities within the insurance sector. Continuous improvement and adaptation of fraud detection methods are crucial as fraud tactics become increasingly sophisticated. Embracing AI offers insurers an opportunity not only to protect their assets but also to heighten the overall customer experience.
To delve deeper into how automation can also enhance claims processes, consider reading our related blog on improving turnaround times through claims automation. For more personalized guidance on implementing AI solutions for enhanced fraud detection, contact us today.