Improving Accuracy with Data Observability in Automated Claims
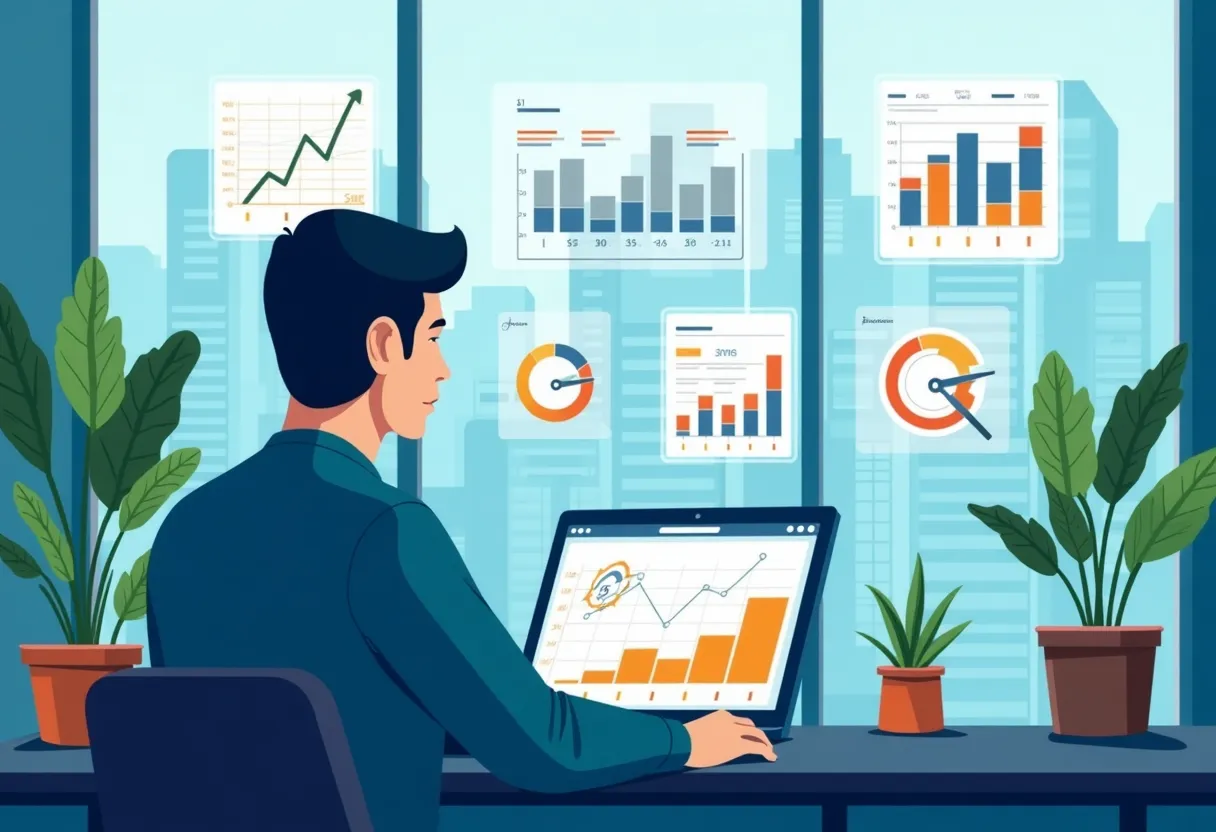
Introduction
In the realm of property and casualty (P&C) insurance, accuracy in automated claims processing is paramount. The nuances of the claims lifecycle demand precision and efficiency to build trust with policyholders and maintain profitability. Thus, achieving high levels of accuracy in claims handling not only enhances customer satisfaction but also mitigates operational risks. Data observability emerges as a critical component in this domain, providing insurers with the insights needed to optimize their automated claims processing systems.
What is Data Observability and Why is it Important for Claims Automation?
Defining Data Observability in the Context of P&C Insurance
Data observability refers to the capability of organizations to understand the state of their data and its flow through various systems. In the context of P&C insurance, it denotes the processes and tools employed to monitor, verify, and troubleshoot the data that fuels automated claims systems. This involves evaluating data quality, identifying discrepancies, and ensuring that accurate information is utilized throughout the claims lifecycle. By maintaining a comprehensive view of data health, insurers can avert errors that could lead to unjust payments or extended claims resolution times.
The Role of Data Observability in Ensuring Accurate Claims Processing
The importance of data observability lies in its preventative nature. By implementing robust observability practices, insurers can avert potential claim disputes and performance bottlenecks before they escalate. Data observability allows for continuous monitoring and real-time insights, helping insurance professionals spot anomalies and data drift that could undermine the accuracy of claims processing. This proactive approach ultimately facilitates a more precise handling of claims, which is essential in today's fast-paced and highly competitive insurance market.
Current Challenges in Claims Processing Without Data Observability
Without data observability, insurers face a myriad of challenges that can hinder the efficiency of their claims systems. Common pitfalls include lack of visibility into data quality, inability to track performance metrics, and delayed identification of errors. These challenges can lead to inflated costs due to inefficiencies, prolonged claims resolution, and most critically, customer dissatisfaction. As such, without a clear understanding of their data ecosystem, insurers may struggle to optimize their operations or respond to changing market demands.
How Does Data Observability Enhance Claims Automation?
Understanding Automated Claims Processing
Automated claims processing refers to the use of systems and technologies designed to handle claims-related tasks with minimal human intervention. This process encompasses a variety of activities, including initial claim intake, documentation review, condition assessment, and payment distribution. Automation improves efficiency and reduces handling times, allowing insurers to manage higher claim volumes without compromising service quality. However, for such systems to function optimally, a foundation of robust data observability must be in place.
Key Features of Data Observability Tools
Data observability tools come equipped with features that significantly enhance claims automation, including:
- Real-time Monitoring: Constant tracking of data flow and integrity allows for immediate detection of anomalies.
- Automated Alerts: Notifications sent to relevant personnel when thresholds are breached ensure swift responses.
- Data Lineage Mapping: Understanding how data moves through systems clarifies potential failure points and improves trust in data quality.
- Integrated Analytics: Advanced analytics identify patterns to support predictive insights and enhance decision-making.
Real-World Examples of Data Observability in Action
Several insurance companies have successfully implemented data observability tools to enhance their claims automation. For instance, a leading insurer utilized observability to streamline the claims process, resulting in a 30% reduction in claim resolution times. By integrating observability, they could pinpoint discrepancies in incoming data, allowing for faster evaluations and reduced fraudulent claims. Such implementations underscore the transformative potential data observability has in driving accuracy and efficiency within automated claims systems.
What Are the Benefits of Implementing Data Observability in Claims Systems?
Improved Accuracy and Reduced Errors
One of the primary advantages of data observability is the marked improvement in accuracy it brings to claims processing. By continuously monitoring data quality and flow, insurers can identify errors and discrepancies before they manifest in claims decisions. This proactive stance in managing data quality reduces the frequency of costly errors that can damage relationships with policyholders and lead to financial losses.
Increased Efficiency and Speed in Claims Processing
Incorporating data observability into claims systems not only enhances accuracy but also boosts overall operational efficiency. With clear visibility into data processes, insurers can streamline workflows, eliminate redundancies, and reduce the time spent on manual checks. This efficiency translates into faster claims processing and a more responsive service, which is vital in meeting the ever-increasing consumer expectations in the digital age.
Enhanced Fraud Detection and Risk Mitigation
The ability to identify anomalies in data patterns significantly enhances fraud detection capabilities. Data observability tools can flag unusual trends or transactions that may indicate fraudulent activities. By implementing these advanced monitoring solutions, insurers can act swiftly to mitigate risks, protect their financial interests, and maintain trust with legitimate policyholders.
How Can Insurers Effectively Adopt Data Observability Tools?
Assessing Current Claims Processing Systems
Before adopting data observability tools, insurers should thoroughly assess their existing claims processing systems. This involves evaluating current workflows, data sources, and pain points that may be hindering efficiency and accuracy. By identifying where data observability can add value, insurers can strategically integrate the tools that best suit their operational needs, leading to a tailored approach that maximizes the benefits of implementation.
Selecting the Right Data Observability Tools
The market offers a diverse array of data observability tools, each delivering unique features and capabilities. Insurers should focus on selecting tools that align with their specific needs, whether it be real-time monitoring, integration capabilities, or advanced analytics. Evaluating vendors based on their support services, scalability, and alignment with industry standards is also critical to ensuring successful adoption.
Best Practices for Integration and Implementation
Successful integration of data observability tools requires careful planning and execution. Insurers should establish a clear roadmap for implementation that includes stakeholder engagement, training programs for users, and phased rollouts to ensure a smooth transition. Continuous feedback and iterative improvements will help fine-tune the utilization of observability tools over time.
What Metrics Should Insurers Monitor Through Data Observability?
Key Performance Indicators (KPIs) for Claims Processing
To gauge the effectiveness of their claims processing systems, insurers must monitor critical KPIs, which can include average claim resolution time, accuracy of claims adjudication, and customer satisfaction ratings. By tracking these metrics, insurers can evaluate the impact of data observability tools on their operations and identify areas for further enhancement.
Monitoring Data Quality and Integrity
Maintaining high data quality and integrity is essential for optimal claims processing. Insurers should monitor data consistency across various systems, ensuring that updates are reflected accurately and promptly. This vigilance is vital to sustaining confidence in the data ecosystem that drives automated claims decisions.
Adapting Metrics Based on Emerging Trends
The insurance landscape is continually evolving, and as such, insurers should remain responsive to emerging trends. This adaptability requires insurers to be proactive in adjusting the metrics monitored by their data observability tools. By aligning their metrics with market developments and consumer behaviors, insurers will be better positioned to maintain accuracy and relevance in their claims processing.
What Are the Future Trends in Data Observability and Claims Automation?
The Impact of Artificial Intelligence and Machine Learning
Artificial intelligence (AI) and machine learning (ML) are set to revolutionize claims automation and data observability in significant ways. These technologies enable insurers to analyze vast datasets rapidly and uncover hidden patterns that can enhance decision-making. As AI and ML tools evolve, they will provide unprecedented levels of insight into claims processing, making it easier for insurers to detect fraud and optimize workflows.
Predictions for the Future of P&C Insurance Claims Processing
As the demand for more efficient and accurate claims processing grows, the integration of data observability with advanced technology will become increasingly dominant. Insurers will likely implement more sophisticated systems that combine observability with predictive analytics, enabling them to anticipate problems and streamline operations preemptively. The fusion of these technologies will ultimately redefine customer experiences and transform the insurance landscape.
Staying Ahead of Industry Changes with Data Observability
For insurers aiming to remain competitive, leveraging data observability is no longer optional; it is essential. As regulatory requirements and consumer expectations evolve, insurers must adapt their strategies to align with these changes. By embracing data observability tools and remaining vigilant in monitoring their data ecosystems, insurers can navigate industry shifts and capitalize on emerging opportunities successfully.
Conclusion
In summary, data observability plays a crucial role in improving accuracy within automated claims processing systems. By providing insights that enhance data quality and operational efficiency, observability not only mitigates errors but also simplifies fraud detection and risk management. In a rapidly evolving insurance landscape, the ongoing necessity for insurers to leverage data observability tools cannot be overstated. For those interested in further exploring the intersection of automation and data in claims management, consider reading our blog on transforming claims management through real-time intelligent automation. To see how Inaza can support your journey in adopting these essential tools, contact us today.