Predictive Analytics: A Game-Changer for Claims Automation
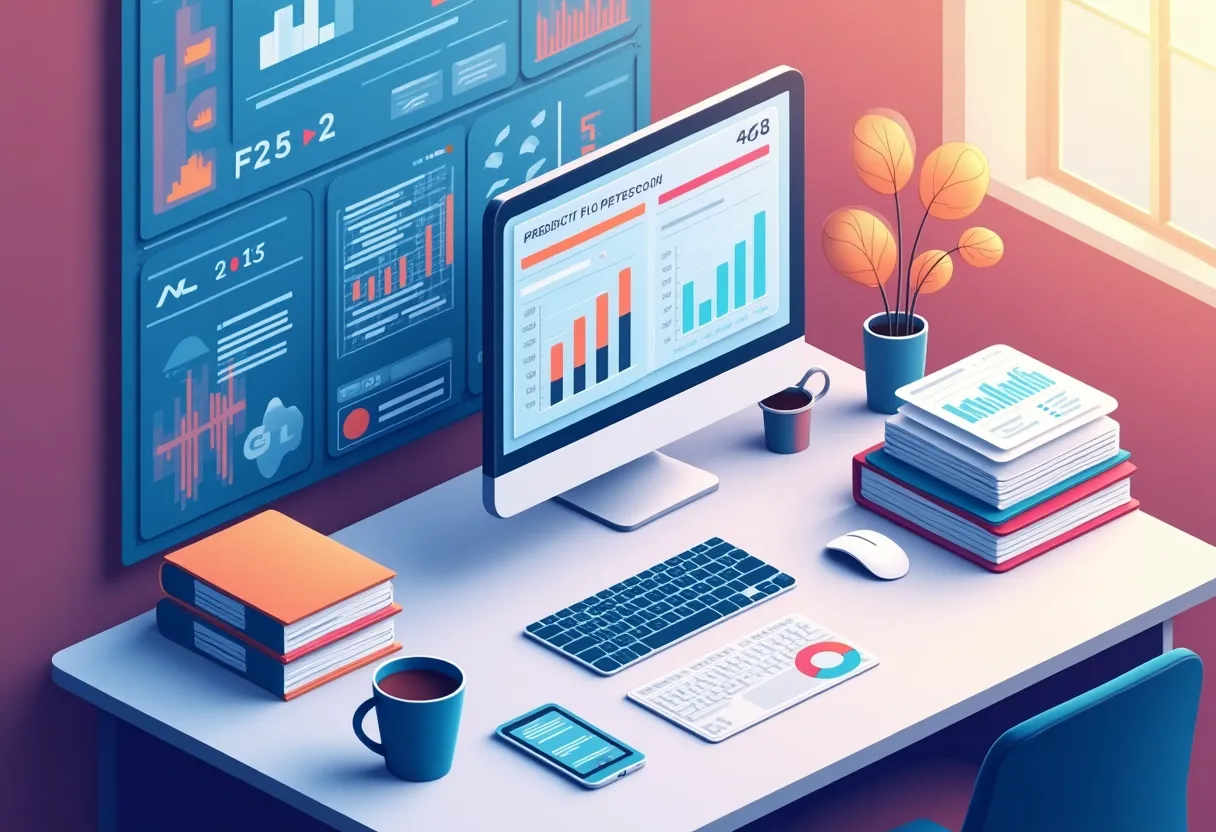
Introduction
Predictive analytics has emerged as a cornerstone of innovation in the insurance industry, revolutionizing how companies approach claims processing and management. As insurers seek to enhance operational efficiency, streamline workflows, and drive customer satisfaction, the integration of predictive analytics into claims automation has become crucial. The ability to analyze vast datasets and generate actionable insights allows insurers to optimize the claims process, ultimately leading to faster resolutions and improved overall service delivery.
By leveraging powerful algorithms and data-driven insights, predictive analytics plays a significant role in automating claims processes. This advancement not only alleviates the administrative burden on claims handlers but also expedites decision-making. As the insurance sector faces increasing pressure to respond to customer needs promptly and effectively, predictive analytics stands out as a game-changing tool that turns data into impactful operational strategies.
How Does Predictive Analytics Enhance Claims Automation?
Understanding Predictive Analytics
At its essence, predictive analytics refers to the systematic approach of using historical data, statistical algorithms, and machine learning techniques to predict future outcomes. It combines various components such as data mining, predictive modeling, and statistical techniques to ascertain patterns that can inform decision-making processes. The underlying principle is to utilize existing data to build a comprehensive model that anticipates potential future scenarios.
Data mining is a critical component of predictive analytics, operating within a framework that identifies patterns and relationships in extensive datasets. By employing sophisticated statistical algorithms, insurers can derive insights regarding factors influencing claims approval, fraud detection, and other key areas relevant to claims automation. This predictive capability transforms claims handling from a conventional process to an advanced, data-centric function.
Predictive Analytics in Claims Processing
In the implementation of claims processing, predictive analytics serves as a vital element that enhances decision-making. By utilizing predictive models, insurers can evaluate claims more effectively – determining the likelihood of legitimacy, potential fraud, and standard processing times. The predictive capabilities allow insurers to automate routine tasks, minimizing human error and improving operational performance.
Successful case studies illustrate the effectiveness of predictive analytics in improving claims operations. For instance, insurance companies that have adopted these techniques report significantly reduced claim processing times and enhanced accuracy in the assessment of claims. Claims automation not only simplifies the administrative flow but also fosters a culture of continuous improvement, ensuring that companies can scale operations without sacrificing quality.
What Are the Benefits of Using Predictive Analytics in Claims Automation?
Improved Efficiency and Speed
One of the most notable advantages of predictive analytics in claims automation is the marked improvement in efficiency and speed. By automating repetitive tasks typically associated with claims handling, insurers can significantly reduce processing times. Predictive modeling enables expedited workflows, ensuring that claims are evaluated swiftly and accurately.
This enhanced speed translates to quicker resolutions for claimants, which is essential in a competitive insurance landscape. Clients today expect timely and efficient service, and predictive analytics meets this demand, allowing insurers to streamline operations while maintaining high levels of customer satisfaction.
Enhanced Accuracy in Claims Assessment
The application of predictive analytics also results in improved accuracy in claims assessment. By leveraging data and statistical algorithms, insurers can minimize human errors that may occur during the claims evaluation process. Historical data from previous claims provides a basis for more precise assessments, allowing underwriters to make informed decisions based on case profiles and associated risks.
This level of accuracy ensures that legitimate claims are processed without unnecessary delays, while at the same time flagging potentially fraudulent ones for further investigation. By utilizing predictive analytics in claims management, insurers can confidently ensure that they address claims systematically based on data-driven insights.
Cost Savings Through Automation
Implementing predictive analytics also bears financial implications, leading to significant cost savings for insurers. By automating processes and improving the overall efficiency, companies can allocate resources more strategically, thus minimizing operational costs. Furthermore, the reduction in fraudulent claims through advanced detection systems means that potential losses are curtailed, contributing to overall savings.
Long-term cost reductions stem not only from operational efficiencies but also from the reduction of fraud losses. Predictive analytics enables insurers to proactively combat fraudulent activity, thereby preserving essential capital and strengthening their financial footing in an increasingly competitive market.
How Does Predictive Analytics Help Identify Fraudulent Claims?
Identifying Patterns in Claims Data
One of the most powerful applications of predictive analytics is its ability to identify patterns within claims data that may indicate fraudulent activity. By analyzing historical claims data, predictive models can detect anomalies that differ from the norm, such as unusual spikes in claim frequency or discrepancies in reported damages.
The importance of historical data analysis cannot be overstated; it serves as a reference point that allows insurers to distinguish between regular claims activities and those that raise suspicions. Through sophisticated data analysis techniques, predictive analytics can identify behavioral patterns associated with fraudulent claims, allowing insurers to take proactive measures to investigate and mitigate risk.
Real-Time Monitoring Capabilities
Beyond identifying patterns, predictive analytics also equips insurers with real-time monitoring capabilities. Automated tools can flag suspicious claims as they occur, enabling swift intervention and investigation. Timely identification of potential fraud is crucial; it allows claims handlers to address questionable submissions before they escalate into larger financial losses.
With real-time data-harnessing tools, insurers can build more resilient systems capable of responding to fraud attempts effectively. The marriage of predictive analytics and automation facilitates a proactive stance against potential threats, ensuring that insurers remain one step ahead in the fraud detection landscape.
What Challenges Do Insurers Face When Implementing Predictive Analytics?
Data Privacy and Security Concerns
While the benefits of predictive analytics are significant, insurers must navigate various challenges when implementing these technologies. Data privacy and security concerns top the list, especially as regulatory compliance requirements evolve. Insurers must handle sensitive customer information responsibly while ensuring they meet the legal implications surrounding data usage.
Measures to protect sensitive information necessarily include implementing robust data governance frameworks, investing in secure technologies, and ensuring staff are trained in data handling best practices. Without adequate measures, insurers risk severe penalties and reputational damage that can hinder their operational success.
Integration with Existing Systems
Another significant challenge lies in integrating predictive analytics with existing legacy systems. Many insurance organizations struggle to merge new technologies with outdated infrastructures, resulting in inefficiencies or incomplete implementations. A successful integration strategy must account for existing workflows and technology performance, ensuring that adaptations do not disrupt service continuity.
To effectively implement predictive analytics, insurers may need to invest in technology upgrades, create robust APIs for data exchange, and develop change management plans that facilitate smooth transitions between old and new systems.
Change Management and Workforce Training
Lastly, change management and workforce training present challenges to organizations adopting predictive analytics. The introduction of new technologies often meets resistance from employees who may be accustomed to traditional workflows. Training staff to utilize predictive analytics effectively and addressing their concerns are critical for overcoming resistance.
Insurers can create a culture of innovation by providing ongoing education about the benefits of predictive analytics and illustrating its potential for enhancing workflow efficiency. Involving employees in the implementation process fosters acceptance and paves the way for smoother transitions.
What Does the Future Hold for Predictive Analytics in Claims Automation?
Innovations on the Horizon
As technology continues to advance, predictive analytics is set to evolve further, unlocking new dimensions for its application in claims automation. Emerging technologies, such as Internet of Things (IoT) devices and augmented analytics, are anticipated to reshape how data is collected and analyzed, setting the stage for more refined predictive models.
Future trends indicate a shift toward more integrated systems that can seamlessly connect various data sources, enhancing the accuracy and reliability of insights derived from predictive analytics. The insurance landscape is poised for unprecedented growth and transformation driven by these innovations.
The Evolving Role of AI in Claims Automation
Artificial intelligence (AI) will play a pivotal role in the future of predictive analytics, propelling it to new heights of sophistication. The compatibility between AI technologies and predictive analytics allows for sophisticated algorithms to process data in real-time, enhancing efficiency and accuracy in claims processing.
AI-driven predictive analytics can improve decision-making further by identifying emerging trends in claims data and suggesting proactive interventions. As insurers continue to embrace AI technologies, the implications for claims automation hold promise for substantial advancements in operational capabilities.
Conclusion
The transformative role of predictive analytics in claims automation is evident in the critical improvements it offers in efficiency, accuracy, and cost-savings, all while fortifying fraud prevention efforts. As insurance companies navigate a competitive landscape, embracing such advancements positions them not just to survive but to thrive in a rapidly evolving market.
For insurers looking to remain ahead in adopting these powerful technologies, understanding the future trends and potential innovations can guide strategic decisions. For a deeper look at insurance innovations, consider exploring how intelligent automation is reshaping the FNOL processes in our related blog: how intelligent automation is revolutionizing FNOL processes. If you're ready to enhance your claims automation strategy and leverage predictive analytics effectively, contact us today.