Predictive Analytics and Its Role in Preventing Insurance Fraud
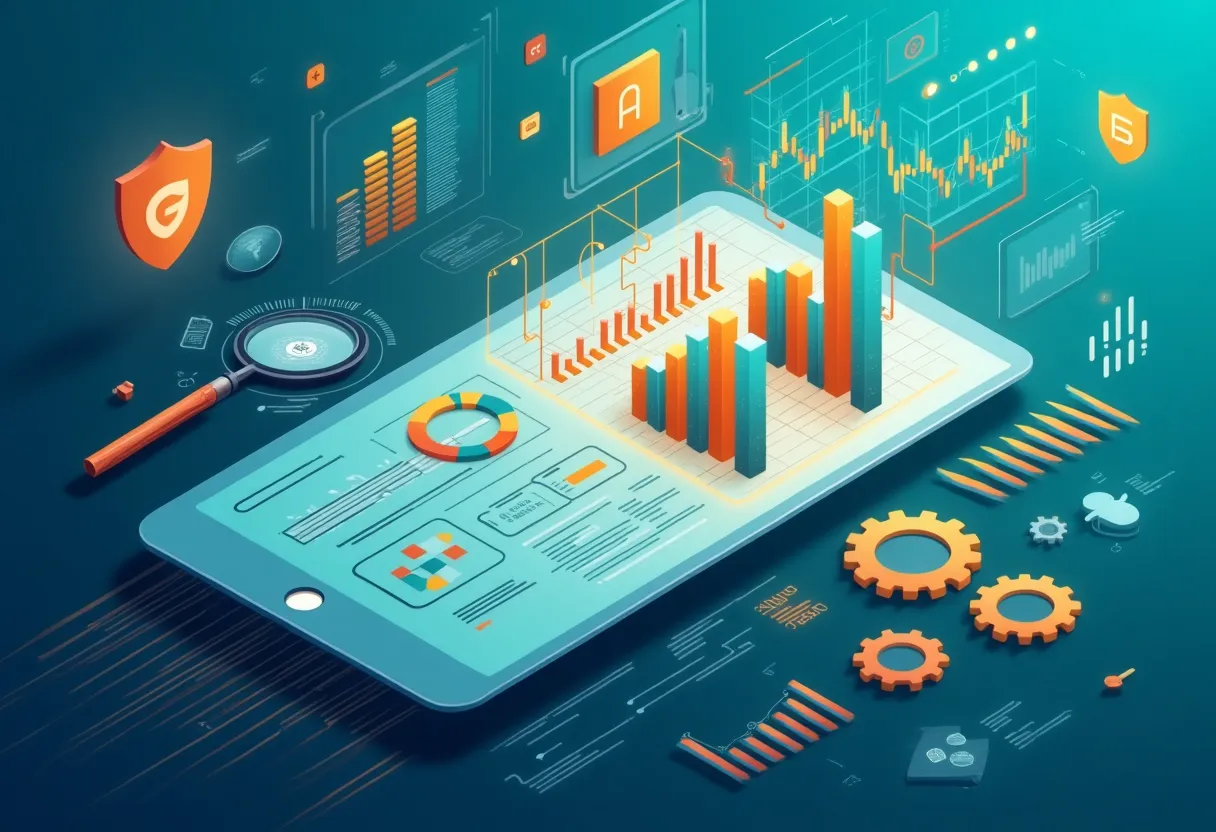
Introduction
Insurance fraud represents a significant challenge for the sector, costing billions each year and eroding the trust between insurers and policyholders. This crime manifests in various forms, from exaggerated claims to outright fabrication. Beyond the immediate financial losses, fraud contributes to inflated premiums and operational inefficiencies, impacting all stakeholders. As the insurance landscape becomes increasingly complex, proactive measures for fraud detection are more crucial than ever.
To combat the rising tide of fraudulent activities, the insurance industry is turning to innovative technologies, notably predictive analytics. By leveraging vast datasets and advanced algorithms, predictive analytics offers a robust solution for identifying and preventing fraudulent claims before they are paid out.
What is Predictive Analytics and Why Does it Matter in Insurance Fraud Detection?
Understanding Predictive Analytics
Predictive analytics involves the use of statistical algorithms and machine learning techniques to identify the likelihood of future outcomes based on historical data. In the realm of insurance, this translates to analyzing claims histories, customer behaviors, and various external data points to predict potential fraud. By utilizing these insights, insurers can make more informed decisions, allocating resources where they are needed most.
The beauty of predictive analytics lies in its ability to process large volumes of data swiftly, uncovering hidden patterns and correlations that may not be apparent through traditional analysis. This capability allows insurers to move from reactive to proactive strategies, making significant strides in combating fraud.
Key Techniques Used in Predictive Analytics
Predictive analytics uses several key techniques, including regression analysis, decision trees, and neural networks. Regression analysis can determine how different variables affect claim outcomes; decision trees provide a visual representation of decision-making processes, and neural networks can model complex relationships in data. By combining these methodologies, insurers can effectively analyze multifaceted patterns indicative of fraudulent behavior.
How Predictive Analytics Differs from Traditional Analytics
Traditional analytics focuses primarily on past performance, evaluating "what happened" rather than "what could happen." In contrast, predictive analytics shifts the focus toward forecasting future events, making it a more effective tool in the fight against insurance fraud. This forward-thinking approach not only enhances detection capabilities but also streamlines operational efficiencies by allowing organizations to allocate resources to high-risk claims.
How Can Predictive Analytics Help Identify Potential Fraudulent Claims?
Identifying Patterns and Anomalies in Data
Predictive analytics excels at recognizing patterns and anomalies within large datasets. For instance, insurers can flag unusual claims that deviate from typical behavior, such as a significantly higher number of claims filed within a short time frame. Machine learning algorithms continuously learn from new data, ensuring that detection systems adapt and evolve, becoming increasingly accurate over time.
This capability is crucial in identifying emerging fraud trends before they escalate into larger issues. By focusing on these anomalies, insurers can investigate suspicious claims preemptively, saving valuable resources and protecting their bottom line.
Risk Scoring: Who Should Be Flagged for Further Review?
Another essential aspect of predictive analytics in fraud detection is the use of risk scoring. This technique allows insurers to assign a numerical score to each claim based on various risk factors identified during analysis. High-risk claims can be flagged for thorough investigation, enabling a targeted approach to fraud prevention.
This method not only enhances operational efficiency but also reduces the burden on claims adjusters who might otherwise be overwhelmed. By focusing on the highest-risk cases, insurers can expedite the review process and minimize fraudulent payouts.
Real-World Examples of Successful Fraud Detection
Many organizations are successfully utilizing predictive analytics to enhance their fraud detection capabilities. For instance, leading insurance companies have adopted AI-driven models that analyze historical claim data and correlate it with external information, such as social media activity and public records. Such comprehensive analysis allows for effective flagging of suspicious claims.
By implementing these advanced techniques, companies have seen significant reductions in fraudulent claims, leading to enhanced profitability and improved customer trust.
What Data Sources Are Utilized in Predictive Analytics for Fraud Detection?
Internal Data: Claims History and Customer Behavior
Internally, insurers rely heavily on claims history and customer behavior data for predictive analytics. This information, accumulated over time, provides invaluable insights into patterns of claim submissions, allowing predictability regarding future behavior. By analyzing historical claims data, insurers can develop profiles of typical claimants, helping to quickly identify those with a history of suspicious activity.
External Data: Public Records and Social Media Analytics
In addition to internal data, external sources such as public records and social media analytics significantly augment fraud detection efforts. Public records can provide information regarding an individual's history and behavior patterns, while social media analytics can reveal inconsistencies in claims, such as lifestyle anomalies that contradict reported injuries. The integration of external data allows for a holistic view of potential fraud risks, drastically enhancing detection accuracy.
The Role of Machine Learning in Enhancing Data Analysis
Machine learning plays a pivotal role in the efficacy of predictive analytics by enabling systems to learn from data and improve over time. Through algorithms that identify complex patterns and relationships, machine learning models can evaluate vast datasets far more efficiently than traditional methods. This advanced analysis not only improves fraud detection rates but also reduces false positives, ensuring that legitimate claims are processed without unnecessary delays.
What Are the Challenges of Implementing Predictive Analytics in Insurance Fraud Detection?
Data Privacy and Ethical Considerations
While predictive analytics offers numerous benefits, it is not without challenges. Data privacy and ethical considerations loom large in the insurance sector. Insurers must navigate regulations concerning the use of personal data to ensure compliance while still leveraging insights offered by predictive analytics. Failing to do so can lead to significant legal ramifications and damage customer trust.
Integrating Predictive Models with Existing Systems
Integrating predictive models with existing claims management systems can also present hurdles. Insurers often operate using legacy platforms that may not easily accommodate the latest technologies. This lack of integration can hinder the overall effectiveness of predictive analytics, making it challenging for organizations to realize the full potential of their fraud detection efforts.
Resistance to Change: How to Overcome Industry Inertia
Lastly, the insurance industry is often characterized by resistance to change. Decision-makers might be hesitant to invest in new technologies or overhaul existing processes. To foster a culture of innovation, it is essential to demonstrate the definitive ROI of predictive analytics and cultivate an environment where continuous improvement is prioritized. Engaging in training and showing benefits through pilot programs can help mitigate resistance and encourage wider adoption.
How Can Insurers Optimize Their Fraud Detection Processes Using Predictive Analytics?
Building a Comprehensive Fraud Detection Strategy
To optimize fraud detection processes, insurers need to develop a comprehensive strategy that effectively integrates predictive analytics into their existing frameworks. This strategy should encompass advanced data analysis techniques, robust training programs for staff, and clearly defined procedures for handling flagged claims. Establishing performance metrics will also help gauge the effectiveness of the predictive models in place.
Training Staff and Promoting a Fraud-Aware Culture
Investing in training programs allows staff to gain essential tools and knowledge regarding the nuances of predictive fraud detection. Fostering a fraud-aware culture ensures that every member of the team understands the importance of vigilance and the role they play in maintaining integrity within the claims process. Such initiatives lead to more effective collaboration across departments and greater overall success in mitigating fraud.
Continuous Monitoring and Model Adjustment
Finally, continuous monitoring and regular adjustment of predictive models are crucial to maintaining their effectiveness. The landscape of insurance fraud is ever-evolving; thus, models need to adapt accordingly. By routinely evaluating and refining these models, insurers can enhance their fraud detection capabilities and stay ahead of emerging trends and tactics used by fraudsters.
What is the Future of Predictive Analytics in the Insurance Industry?
The Impact of Advancements in AI and Machine Learning
The future of predictive analytics in the insurance industry is bright, particularly with advancements in AI and machine learning. These tools are poised to further revolutionize the way insurers detect and prevent fraud. As algorithms become more sophisticated, the predictive capabilities will only improve, potentially leading to a significant decrease in fraudulent claims across the sector.
Innovative Applications Beyond Fraud Detection
Beyond fraud detection, predictive analytics holds the promise of enhancing various facets of the insurance industry. From personalized policy recommendations to optimized pricing models, these technologies can transform customer experiences and operational efficiencies. The ability to anticipate customer needs and market trends will profoundly impact insurers’ strategic approaches moving forward.
Evolving Regulatory Landscape: What Insurers Need to Know
As the insurance industry evolves, so too does the regulatory landscape. Insurers must remain vigilant regarding changes in regulations surrounding data usage and privacy, ensuring their practices align with legal requirements. Staying ahead of regulatory developments will be critical for insurers aiming to leverage predictive analytics effectively without falling afoul of compliance mandates.
Conclusion
In conclusion, predictive analytics plays a pivotal role in preventing insurance fraud, offering a proactive approach that enhances fraud detection and prevention efforts. As insurers increasingly embrace these strategies, they stand to benefit from improved operational efficiency and reduced fraudulent activity. To harness the full potential of predictive analytics, insurers must invest in the necessary technologies, data integration strategies, and staff training programs.
For insurers looking to delve deeper into the innovative applications of technology in insurance, our blog on AI-driven email analytics transforming insurance reporting and audits provides valuable insights. To explore how predictive analytics can revolutionize your fraud detection strategy, contact us today.