The Future of Insurance: Full Stack AI-Powered Predictive Analytics
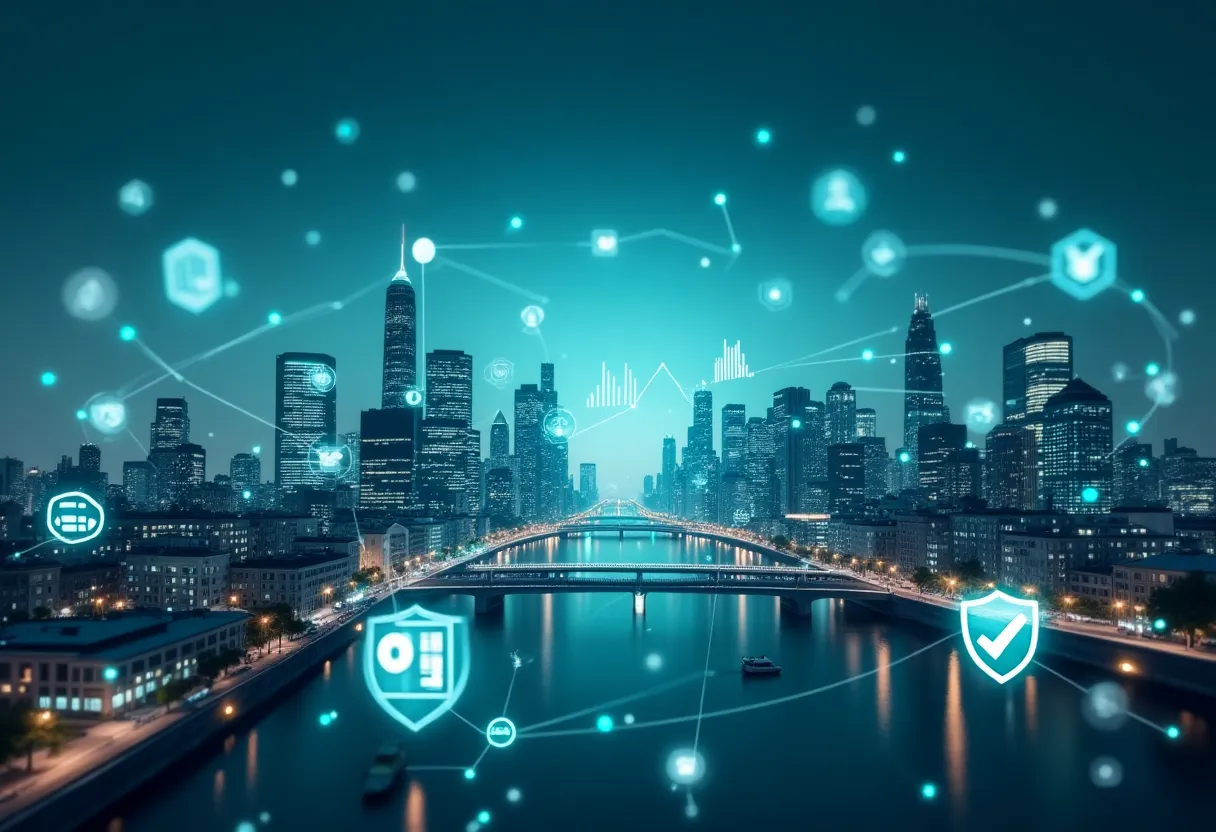
What is Full Stack AI and How is it Revolutionizing the Insurance Industry?
Full Stack AI refers to the integration of artificial intelligence across all layers of technology stacks in an insurance firm, encompassing data collection, processing, analysis, and dissemination. It revolutionizes the industry by enabling insurers to leverage vast amounts of information to drive decision-making processes. With the advent of Full Stack AI, the insurance landscape is shifting from traditional methods to a robust, data-driven, strategic approach where predictive analytics play a key role.
Understanding Full Stack AI
Full Stack AI provides a comprehensive framework that encompasses various AI technologies, including machine learning, natural language processing, and predictive analytics. This all-encompassing approach facilitates streamlined processes, improved accuracy in decision-making, and enhanced customer interactions. Full Stack AI can analyze historical data, recognize patterns, and predict future outcomes, allowing insurance companies to adapt to changing market dynamics effectively.
Differences Between Traditional Analytics and Full Stack AI
Traditional analytics typically rely on static models and predefined parameters, which limit the insights they can offer. In contrast, Full Stack AI employs adaptive algorithms that learn from ongoing data inputs. This fundamental shift allows organizations to avoid the pitfalls of outdated practices and embrace a predictive model that evolves with real-time information, significantly enhancing their operational agility.
Key Components of Full Stack AI in Insurance
Implementation of Full Stack AI in insurance commonly consists of several critical components:
- Data Acquisition: Gathering extensive datasets from various sources.
- Data Processing: Cleaning and organizing data for analysis.
- Predictive Analytics: Applying machine learning algorithms to identify patterns and make predictions.
- AI-Driven Decision-Making: Utilizing insights derived from analytics to inform strategic actions.
These components work synergistically to create a more efficient insurance ecosystem, enabling companies to refine their products and services based on data-driven insights.
How Does Full Stack AI Enhance Risk Prediction?
Risk prediction is paramount in the insurance sector, influencing pricing, underwriting, and overall business strategy. Full Stack AI significantly enhances risk management by leveraging intricate algorithms that analyze past, present, and potential future events.
The Role of Predictive Analytics in Risk Management
Predictive analytics serves as the backbone of risk management in modern insurance practices. Through data modeling and analysis, insurers can identify potential risks before they materialize, thus minimizing losses. For instance, predictive models can highlight which policyholders are more likely to file claims based on historical behavior, enabling companies to adjust premiums accordingly and enhance their risk stratification methods.
Machine Learning Models for Accurate Risk Assessment
Machine learning models are essential for refining risk assessments. By utilizing algorithms that learn from historical data, these models can process complex variables and provide increasingly accurate estimates of risk associated with each policyholder. Their predictive capabilities ensure that insurers stay ahead of potential underwriting challenges, allowing for more granular assessment of risks.
Case Studies: Successful Implementation of Risk Prediction Models
While specific case studies are not included, the general trend shows that leading insurance firms implementing Full Stack AI have significantly advanced their risk prediction capabilities. Through data-driven decision-making, these firms report not only enhanced risk assessment accuracy but also improved customer satisfaction and loyalty.
In What Ways Can Full Stack AI Improve Claims Forecasting?
Claims forecasting plays a crucial role in determining a company’s financial health and operational efficiency. Accurate forecasts allow insurers to allocate resources effectively and predict cash flow needs.
The Importance of Accurate Claims Forecasting
Accurate claims forecasting helps insurers anticipate the volume and severity of claims, aiding in better financial planning and risk management. Understanding future claims trends allows organizations to maintain adequate reserves, ensuring they can meet obligations without impacting liquidity or profitability.
Integrating Predictive Models for a Reliable Claims Process
Integrating predictive models into the claims process enhances reliability and efficiency. These models analyze trends within claims data over time, helping companies foresee potential surges in claims activity stemming from unforeseen events or changes in the marketplace. By proactively addressing these trends, insurers can manage their resources more effectively, resulting in a smoother claims experience for customers.
Machine Learning Techniques in Claims Assessment
Machine learning techniques enhance claims assessments by providing real-time analytics that can identify fraudulent claims quickly and accurately. Utilizing historical claims data, machine learning algorithms can recognize anomalies that deviate from established patterns, enabling quicker investigations and decisions.
How Does AI-Powered Analytics Enhance Claims Accuracy?
Claims accuracy is vital for maintaining trust with clients and ensuring operational viability. AI-powered analytics provide a pathway to improved accuracy in claims handling.
Eliminating Human Error in Claims Processing
One of the most significant advantages of AI in claims processing is its ability to eliminate human error. AI algorithms consistently perform evaluations without the biases or inconsistencies associated with manual analysis. This leads to a more accurate evaluation of claims and fosters trust between insurers and policyholders.
The Contribution of Data Management to Claims Accuracy
Effective data management is essential for enhancing claims accuracy. By maintaining accurate, up-to-date datasets that feed into AI systems, insurers can ensure their analytical outcomes are precise and valid. This data-driven approach allows for better visibility into claims transactions, fostering quicker resolution times and improved service delivery.
Real-World Examples of Enhanced Claims Accuracy Using Full Stack AI
Examples abound where insurers have deployed Full Stack AI to elevate claims accuracy. Utilizing multi-dimensional data analysis and machine learning capabilities, these firms report considerably reduced errors and enhanced operational efficiency in their claims handling processes.
What Role Does Full Stack AI Play in Fraud Detection?
Fraud detection remains a significant challenge within the insurance industry, impacting both profitability and customer trust. Full Stack AI provides formidable tools for addressing these issues.
Understanding Insurance Fraud: Types and Impacts
Insurance fraud can manifest in various forms, including premium fraud, claims fraud, and provider fraud. These deceptive practices not only result in financial losses for insurers but also erode client trust and drive up premiums for honest policyholders.
Leveraging AI for Real-Time Fraud Detection
Full Stack AI leverages machine learning models to analyze claims in real-time, enabling rapid identification of potential fraudulent activities. By recognizing patterns consistent with fraudulent behavior in historical datasets, AI systems can flag suspicious claims for further investigation almost instantaneously, thereby protecting insurers from losses.
Success Stories: How Full Stack AI Detected Fraudulent Claims
While specific success stories are kept to general trends, insurance companies leveraging Full Stack AI report significant reductions in fraudulent claims through the deployment of advanced analytics. By continuously refining their models with fresh data, these firms enhance their ability to detect fraud preemptively and adapt to evolving fraudulent tactics.
What Are the Challenges Associated with Implementing Full Stack AI in Insurance?
Despite its potential, the implementation of Full Stack AI does not come without challenges, spanning technical hurdles and organizational readiness.
Common Misconceptions About AI in Insurance
Common misconceptions about AI in insurance often revolve around fears of automation replacing human jobs or that AI technology is too complicated to implement. In reality, AI serves as a complement to human expertise, enhancing efficiency without sacrificing quality of service. The truth is that embracing AI leads to enhancement in operational effectiveness and quality improvement.
Technical and Organizational Barriers to Adoption
Technical barriers such as legacy systems can hinder the adoption of Full Stack AI. Additionally, organizational resistance to change often stands in the way of smooth integration. Insurers must cultivate a culture that embraces technological evolution alongside investing in training for both staff and the infrastructure necessary for AI deployment.
Solutions for Smooth Integration of Predictive Analytics
Organizations can ease the transition into Full Stack AI by starting with pilot projects, investing in employee training, and ensuring robust data governance frameworks. By fostering a culture open to innovation, insurers can leverage predictive analytics effectively and foster buy-in from all stakeholders.
How Will Full Stack AI Shape the Future of the Insurance Industry?
The future of the insurance industry will undoubtedly be influenced heavily by advancements in Full Stack AI. As more organizations adopt these technologies, they will benefit from enhanced insights into customer behavior, risk, and claims management.
Emerging Trends in AI and Insurance
Emerging trends suggest that AI will increasingly enable personalization in insurance policies through data-driven insights. Predictive models will be crucial for customizing offerings based on individual customer profiles, ensuring better alignment with market demand.
Predictions for the Next 5-10 Years
In the next 5-10 years, the integration of Full Stack AI within the insurance industry is expected to deepen. This evolution will likely yield enhanced operational efficiencies, improved customer experiences, and heightened competitiveness among firms leveraging these advanced technologies.
Preparing for the AI-Driven Future in Insurance
Preparing for an AI-driven future requires insurers to invest in technology, train personnel, and cultivate a culture that prioritizes innovation and flexibility. Collaboration with technology partners can also drive the adoption of AI solutions tailored to unique business needs and objectives.
Conclusion: Embracing Full Stack AI for a Transformative Future in Insurance
In summary, Full Stack AI presents an unprecedented opportunity for insurers to revolutionize their operations. By embracing predictive analytics and machine learning, companies can enhance their risk prediction capabilities, improve claims accuracy, and effectively detect fraud. Staying ahead in technology is essential for maintaining competitiveness, and organizations must leverage these advancements to increase efficiency and customer satisfaction.
To discover more about how our solutions can help you harness Full Stack AI, visit our comprehensive insights on Full Stack AI-powered predictive analytics. If you're interested in implementing these transformative technologies, contact us today.