The Role of AI in Automating Multi-Channel Insurance Data Analysis
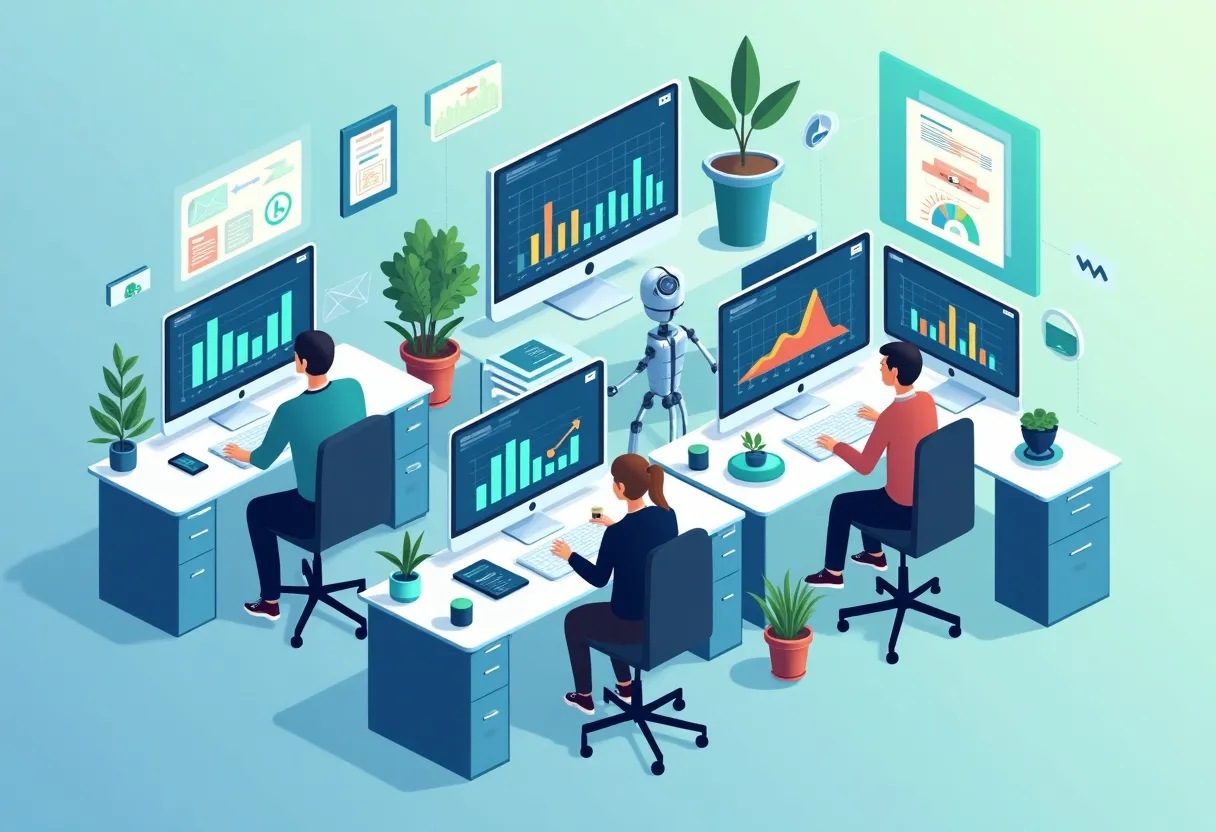
What is Multi-Channel Insurance Data Analysis and Why is it Important?
Multi-channel insurance data analysis refers to the process of gathering, examining, and leveraging data from various sources and platforms—such as social media, mobile apps, websites, and customer service touchpoints—to optimize insurance operations. This analytical approach allows insurers to gain a comprehensive view of their business environment, customer behaviors, and operational challenges. By integrating data from multiple channels, insurers can make informed decisions that enhance service delivery and policy management.
Definition and Scope of Multi-Channel Data Analysis
The scope of multi-channel data analysis in insurance encompasses a wide range of data types, including structured data from policy transactions, unstructured data from customer interactions, and semi-structured data from online inquiries and social media engagements. The analysis can include assessing customer preferences, claims histories, underwriting data, and market trends, employing techniques that transform raw data into actionable insights. This synergy across different data streams paves the way for creating personalized insurance products and targeted marketing strategies that align with consumers' needs.
The Importance of Data in Insurance Operations
Data plays a critical role in insurance operations as it acts as the cornerstone for decision-making. Accurate and timely data facilitates better risk assessment, enhances customer experience, and enables the creation of competitive pricing models. Furthermore, with the rise of digital transformation, insurers are compelled to derive insights that not only respond to market demands but also predict future trends. Efficient data management can lead to improved operational efficiency, reduced overhead costs, and enhanced revenue generation.
Overview of Industry Challenges in Data Management
Despite its significance, the insurance industry faces numerous challenges in managing multi-channel data. These challenges include data silos, where information is trapped within specific departments or platforms, and difficulties in ensuring data quality and integrity. Additionally, numerous legacy systems hinder insurers from effectively harnessing the full potential of their data. As a result, many organizations struggle to employ a unified strategy for data analysis, which can lead to missed opportunities for customer engagement and risk management.
How Does AI Enhance Efficiency in Insurance Data Analysis?
Artificial Intelligence (AI) significantly enhances efficiency in insurance data analysis by automating routine tasks that would otherwise consume valuable human resources. Through advanced algorithms, AI swiftly processes vast amounts of data, enabling insurers to derive insights faster than traditional methods. This acceleration not only improves operational workflow but also allows businesses to focus on strategic initiatives rather than getting bogged down in data minutiae.
Streamlining Data Collection Across Channels
AI simplifies the data collection process by employing bots and advanced data scraping techniques to gather information from various channels seamlessly. Automating this process reduces manual entry errors and ensures that data remains current and reliable. Moreover, the ability to collect data in real-time allows insurers to respond promptly to changing market conditions and customer preferences, thereby enhancing the overall customer experience.
Automation of Data Processing and Reporting
Once data is collected, AI continues to play a vital role by automating the data processing and reporting phases. Utilizing intelligent algorithms, AI can quickly classify, analyze, and visualize data, transforming complex datasets into clear insights. Automated reporting features eliminate time-consuming tasks, allowing teams to focus their efforts on analysis and interpretation, ultimately leading to more strategic decision-making.
Temporal and Cost Benefits of AI-Driven Analysis
Implementing AI for data analysis introduces significant temporal and cost efficiencies. By expediting data processing times and minimizing the need for manual oversight, insurers can significantly reduce operational costs while increasing output quality. The AI-driven approach allows insurance companies to allocate resources more effectively, ultimately enhancing profitability and competitiveness. The combination of faster turnaround times and reduced costs makes AI an invaluable asset in today’s fast-paced insurance market.
In What Ways Does AI Improve Risk Assessment in Insurance?
AI greatly enhances risk assessment capabilities, providing insurers with tools that offer deeper insights into potential risks and enabling more informed underwriting decisions. Machine learning techniques allow for the continuous improvement of risk assessment models, which can proactively identify and mitigate risks before they manifest.
Enhanced Predictive Analytics through Machine Learning
Predictive analytics powered by machine learning grants insurers the ability to forecast risk with remarkable accuracy. By analyzing historical data alongside current trends, insurers can identify patterns that suggest higher risks and make adjustments to policy terms accordingly. Such advanced analytics facilitate not just reactive measures but also proactive strategies that align with emerging risk landscapes.
Real-Time Data Monitoring and Risk Identification
AI's capability for real-time data monitoring means that insurers can continuously assess risk exposure as new data becomes available. This immediacy allows for timely interventions should risk factors change, ensuring that insurance companies are always aligned with the actual risk profiles of their clients. Moreover, this continuous loop of data feedback enriches risk models, making them more robust over time.
Case Studies: Successful AI Deployment in Risk Underwriting
While specific case studies are not detailed here, numerous insurers have successfully implemented AI technologies to improve their underwriting processes, showcasing tangible benefits such as improved accuracy in risk evaluation and significantly reduced turnaround times for policy issuance. These implementations highlight the effectiveness of AI in refining underwriting practices through data-driven insights.
How is AI Ensuring Greater Accuracy in Claims Processing?
AI is revolutionizing claims processing by enhancing accuracy and reducing the potential for human error. Automation in claims management ensures that processes are executed consistently and efficiently, resulting in quicker resolutions and improved customer satisfaction.
Reducing Human Error through Automated Algorithms
By deploying automated algorithms within the claims processing workflow, insurers can minimize human errors that might occur during data entry or evaluation stages. This increased precision ensures that claims are assessed based on complete and accurate information, leading to fairer outcomes for both the company and policyholders. The automation streamlines workflows, allowing claims specialists to concentrate on critical thinking tasks and customer interactions.
AI's Role in Fraud Detection and Prevention
AI technologies are instrumental in combating insurance fraud, a significant concern within the industry. Through advanced pattern recognition and anomaly detection, AI systems can identify suspicious activities and flag potential fraud in real-time. By analyzing various data sources—claims history, customer behavior, and social media—AI enhances the insurer's ability to prevent fraudulent claims and uphold the integrity of the insurance process.
Real-World Examples of AI Enhancing Claims Accuracy
While specific examples are not provided, insurers harnessing AI for claims processing have reported notable increases in claim accuracy and efficiency. The ability to process and validate claims quickly while reducing errors has proven to enhance the overall claims experience for consumers.
What are the Key Technologies Driving AI in Insurance Data Analysis?
Several core technologies underpin the success of AI in automating insurance data analysis, each contributing to improved insights and operational performance.
Big Data Technologies: Harnessing Vast Data Sets
Big data technologies allow insurers to manage vast and complex datasets from diverse sources. These technologies facilitate the accumulation and analysis of structured and unstructured data, enabling insurers to derive actionable insights that inform decision-making processes. The effective integration of big data solutions is crucial in capitalizing on the vast amounts of information available in the modern insurance landscape.
AI Data Enrichment and Its Role
AI data enrichment involves enhancing existing datasets with additional context or information to bolster analysis. By integrating third-party data sources, insurers can gain deeper insights into customer behavior and risk factors. This enrichment not only improves the accuracy of predictions and assessments but also allows for a much more nuanced understanding of each policyholder’s unique circumstances.
Cross-Channel Analytics: Integrating Data for Holistic Insights
Cross-channel analytics involves synthesizing data from various channels to paint a complete picture of customer interactions and business performance. By combining insights gathered from mobile apps, social media, and traditional channels, insurers can develop strategies that enhance customer engagement and operational efficiency. This holistic approach to data analytics ensures that insurers remain agile and responsive to emerging market trends.
How are Insurance Companies Adopting AI for Multi-Channel Data Analysis?
The adoption of AI in multi-channel data analysis is gaining momentum within the insurance industry. Various players are leveraging state-of-the-art technologies to stay competitive in a rapidly evolving market.
Current Trends in AI Adoption within the Insurance Sector
Insurers are increasingly adopting AI technologies to facilitate various functions, from customer service enhancements to operational efficiencies. Natural language processing is being used to enhance chatbots, while machine learning models are being applied in underwriting and claims processing. The trend toward digitization ensures that insurers can meet consumer demands for faster, more personalized services.
Case Examples: Insurers Leading the Way in AI Implementation
Prominent insurers are investing in AI technologies to bolster their analytical capabilities. These industry leaders demonstrate improvements in efficiency and customer satisfaction through streamlined processes and enhanced risk assessments. While specific company examples aren't provided here, the general trend indicates a strong commitment to leveraging AI as a strategic priority within the industry.
Challenges and Considerations in Adoption
Adopting AI, however, is not without challenges. Insurers must consider the integration of new technologies with existing systems, data privacy concerns, and the need for staff training. Moreover, organizations have to ensure that their AI models are transparent, ethical, and compliant with industry regulations. Understanding these challenges is crucial for insurers as they navigate the landscape of technological transformation.
What Should Insurers Consider When Implementing AI Solutions?
When implementing AI solutions, insurers must take a structured approach to ensure successful outcomes. Establishing clear objectives and metrics for performance is vital, guiding the AI deployment process effectively.
Establishing Clear Objectives and KPIs
Setting clear objectives around AI implementation is critical. Insurers need to determine what problems they are solving and how success will be measured through key performance indicators (KPIs). This framework allows businesses to quantify the impact of AI solutions and make necessary adjustments along the way.
Choosing the Right Technology Stack
Choosing the appropriate technology stack is another essential consideration. Insurers should evaluate various AI tools and platforms that align with their strategic goals. The right technology will streamline data handling and facilitate the integration of AI-driven solutions into their existing workflows.
Ensuring Data Privacy and Compliance
Data privacy regulations must be a top priority for insurers when adopting AI solutions. Compliance with standards such as the General Data Protection Regulation (GDPR) is essential to protect consumer data and build trust. Insurers need to implement robust data governance frameworks that ensure safe data usage and informed consent from policyholders.
What Does the Future Hold for AI in Insurance Data Analysis?
The future of AI in insurance data analysis looks promising, characterized by continuous innovation and evolution. Emerging technologies are set to reshape the insurance landscape and enhance operational capabilities.
Predictions for AI Technologies in Insurance
As AI technologies advance, we can expect increased personalization in insurance offerings, driven by real-time data analysis. Predictive analytics will become even more sophisticated, offering insurers greater clarity into potential risks and customer preferences. The shift toward a more automated insurance landscape will enable more efficient and tailored services, enhancing both customer satisfaction and operational efficiency.
The Evolving Role of Insurers in an AI-Driven Landscape
Insurers will increasingly act as data-driven decision-makers in an AI-driven ecosystem. The ability to analyze vast amounts of information quickly will empower insurers to mitigate risk proactively and enhance their product offerings. This transformation will allow for a more strategic approach to customer engagement, resulting in more meaningful interactions and improved customer loyalty.
Importance of Continuous Learning and Adaptation
As AI continues to evolve, insurers must embrace a culture of continuous learning and adaptation. Engaging with new developments in technology and changing customer expectations will be key to maintaining competitive advantage. Organizations must invest in ongoing training and development for their teams to stay abreast of industry advancements.
Conclusion
In conclusion, AI plays a transformative role in automating multi-channel insurance data analysis by enhancing operational efficiency, improving risk assessment accuracy, and advancing claims processing accuracy. The integration of AI not only streamlines processes but also provides insurers with actionable insights necessary to stay competitive in a fast-evolving environment. By implementing AI-focused strategies, insurance companies can ensure they are prepared for future challenges and opportunities. To learn more about how AI can redefine your insurance operations, explore our Decoder platform. For personalized guidance on embracing AI solutions, contact us today.