The Role of AI in Fraud Detection for Underwriting Automation
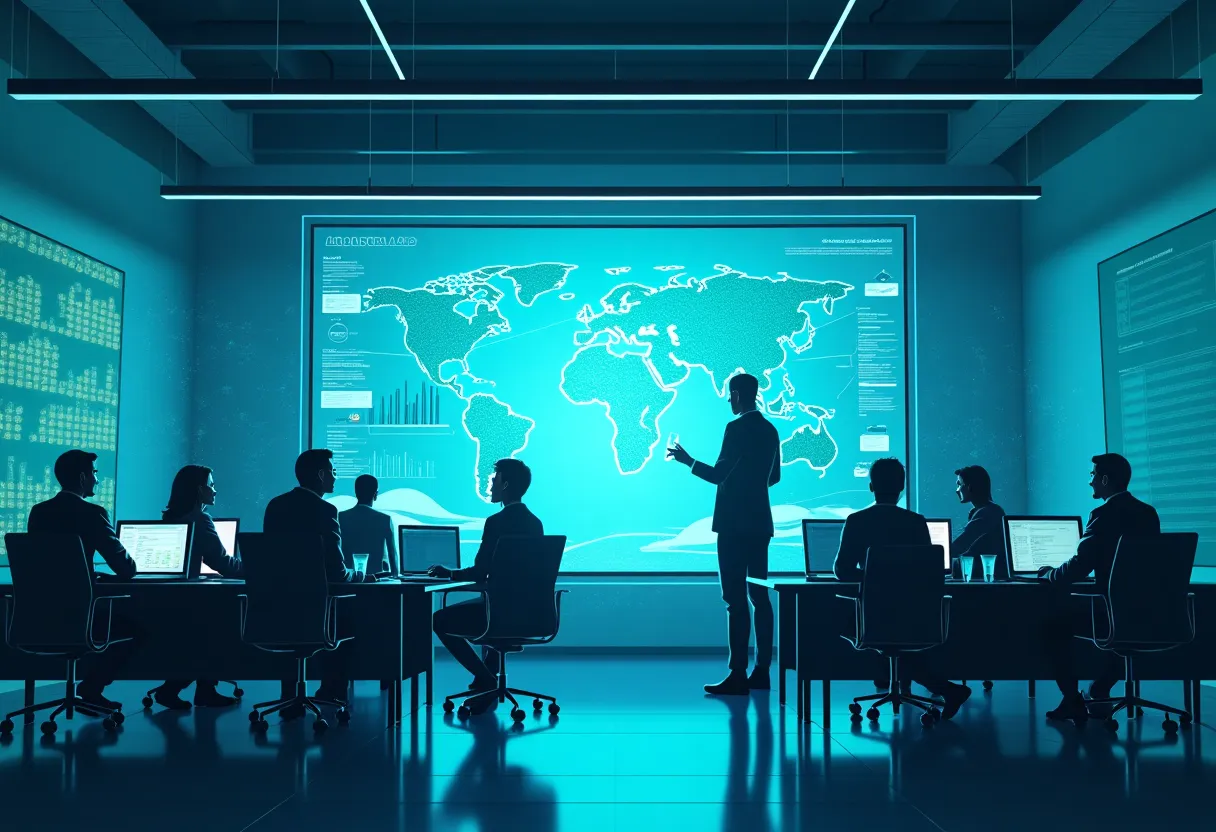
Introduction
In the ever-evolving landscape of insurance, fraud detection in underwriting has become increasingly critical. The potential losses stemming from fraudulent activities not only impact an insurer's financial health but also erode customer trust and damage brand reputation. This challenge has propelled insurers to seek solutions that leverage technology, especially artificial intelligence (AI), to enhance their underwriting processes. By automating fraud detection, insurers can mitigate risks more efficiently and accurately, resulting in significant operational improvements.
Why Is Fraud Detection Crucial in Underwriting Automation?
What Are the Consequences of Fraud in Underwriting?
Fraudulent activities in underwriting can have dire financial implications for insurers. Losses resulting from fraudulent claims can accumulate rapidly, impacting overall profitability and potentially leading to higher premiums for legitimate customers. Furthermore, the reputational consequences of fraud can be long-lasting, as customers are less likely to trust an insurance provider perceived as lenient towards fraudulent activities. This erosion of customer confidence can harm the insurer’s market position and lead to a decline in new business opportunities.
How Does Underwriting Automation Enhance Fraud Detection?
Automated workflows in underwriting allow for the implementation of advanced algorithms that analyze a vast array of data points. By integrating fraud detection systems within these automated processes, insurers gain the ability to evaluate claims against established parameters and patterns of suspicious behavior. Automation also facilitates real-time monitoring of data, enabling companies to identify red flags quickly and respond proactively. This rapid analysis minimizes the likelihood of fraudulent claims being processed, saving the insurer both time and money.
What Types of Fraud are Commonly Encountered in Underwriting?
In the property and casualty insurance sector, there are several types of fraud that underwriters frequently encounter. Common tactics include:
- False Claims: Claimants may fabricate incidents or exaggerate damages to obtain financial gains.
- Staged Accidents: Individuals might stage accidents to generate claims, often involving collusion with others.
- Application Fraud: Misrepresentation of personal information or risk factors during policy application can lead to claims that would otherwise be denied.
How Does AI Transform Fraud Detection in Underwriting Automation?
What AI Technologies Are Used in Fraud Detection?
AI technologies, particularly machine learning algorithms, play a vital role in fraud detection by identifying patterns and anomalies within large datasets. Machine learning can evaluate historical data to recognize behaviors typical of both legitimate and fraudulent claims. Additionally, natural language processing can be employed to analyze unstructured data, such as comments from claimants, allowing systems to assess claims holistically.
How Does AI Improve Data Analysis and Insights?
The ability of AI to process vast quantities of data rapidly enhances the insurer's capacity to detect anomalies. By analyzing underwriting applications in real time, AI systems can flag inconsistencies that may indicate fraudulent behavior. For example, discrepancies between provided information and historical data can prompt further investigation, streamlining the underwriting process while ensuring that risks are managed effectively.
In What Ways Can Predictive Analytics Help Anticipate Fraudulent Activities?
Predictive analytics relies on historical data to identify trends that could signal potential fraud. By evaluating patterns over time, insurers can develop predictive models that gauge the likelihood of fraud based on specific risk factors. This proactive approach allows underwriters to scrutinize high-risk applicants more thoroughly, improving the overall integrity of underwriting processes and minimizing potential losses.
What Are the Benefits of AI-Driven Fraud Detection in Underwriting Automation?
Increased Operational Efficiency: How Does AI Enhance Workflow?
AI-driven fraud detection automates routine tasks and facilitates quicker decision-making. By reducing the manual effort required to screen applications, insurers can focus their resources on more complex cases. This increase in efficiency not only accelerates the underwriting process but also maintains accuracy in risk assessment, enabling insurers to adapt to market demands more robustly.
Improved Accuracy: How Does AI Reduce False Positives?
Enhanced accuracy in fraud detection means that AI systems can distinguish between genuine claims and fraudulent ones with greater precision. Advanced algorithms filter out legitimate applications while flagging those that require further review. This reduction in false positives is crucial, as it minimizes unnecessary delays in processing valid claims, enabling insurers to maintain positive customer relationships.
Enhanced Customer Experience: What Role Does AI Play?
Improved turnaround times facilitated by AI-driven processes result in expedited decision-making, which enhances the customer experience. Clients appreciate faster responses and resolutions, leading to increased satisfaction and loyalty. By balancing fraud prevention with high levels of customer service, insurers position themselves favorably in a competitive marketplace.
What Challenges Might Insurers Face When Implementing AI in Fraud Detection?
Data Privacy and Security Concerns: How to Address These Issues?
The integration of AI into fraud detection must be conducted with stringent data privacy standards. Insurers must comply with regulations such as GDPR and other privacy laws to safeguard sensitive customer data. Strategies must be implemented to anonymize data and ensure that sensitive information is only accessed by authorized personnel.
What Is the Learning Curve for Insurers Adopting AI Technologies?
The shift towards AI-driven systems often entails significant investment in technology, training, and change management. Insurers may face resistance from employees who are accustomed to traditional processes. Effective change management strategies, including comprehensive training and clear communication of AI's benefits, are vital for smooth transitions.
How Can Insurers Ensure Ethical AI Usage?
Insurers must remain vigilant about potential biases in AI algorithms that could lead to unfair treatment of certain applicants. Establishing transparency in AI operations and continuous monitoring are essential components of ethical AI usage. This commitment to fairness is crucial in fostering trust among both employees and customers.
What Future Trends in AI and Fraud Detection Should Insurers Watch For?
How Will Advancements in AI Impact Fraud Detection?
Emerging technologies, such as deep learning and advanced neural networks, hold the potential to revolutionize fraud detection in underwriting. As AI systems become more sophisticated, their ability to analyze data and predict fraud patterns will improve, leading to more robust protective measures against fraudulent claims.
What Are the Implications of a More Connected World on Insurance Fraud?
The growing prevalence of the Internet of Things (IoT) enables more accurate data collection and monitoring, directly influencing fraud detection efforts. Accessing real-time data from connected devices enhances the underwriting process and allows for quicker identification of inconsistencies or suspicious activities. Integrating this diverse data landscape can lead to improved risk assessments and fraud mitigation strategies.
How Can Collaboration Enhance Fraud Prevention Strategies?
Insurers can significantly bolster their fraud detection capabilities by collaborating with industry peers and technology firms. By sharing intelligence on fraudulent activities and employing collective data analytics, companies can strengthen their defenses against fraud. Establishing partnerships across the insurance ecosystem also fosters innovation, enabling the development of more advanced fraud detection solutions.
Conclusion
As AI continues to shape the future of the insurance landscape, its impact on fraud detection within underwriting automation cannot be overstated. By embracing AI-driven strategies, insurers can enhance their ability to prevent fraud while improving efficiency and accuracy in underwriting processes. Adopting these technologies is no longer an option; it is a necessity to safeguard financial stability in an increasingly complex environment. For insurers looking to delve deeper into the transformative effects of data, our previous blog on how big data in the insurance sector is reshaping the market offers insightful perspectives. You can read it here.
To stay ahead of fraudulent activities and revolutionize your underwriting processes, contact us today or book a demo to see how Inaza can assist you in integrating these advanced AI capabilities seamlessly into your operations.