Why Full Stack AI is Revolutionizing Fleet Insurance and Risk Management
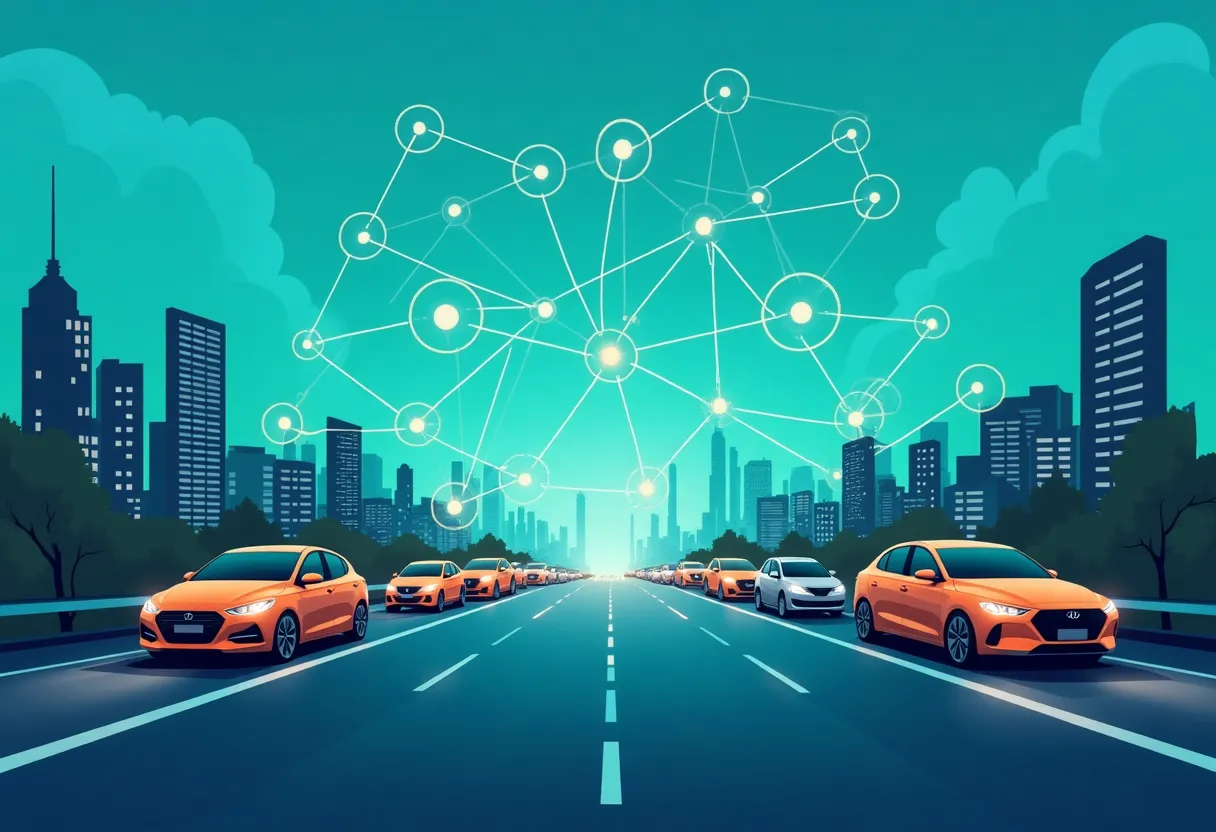
Introduction
The landscape of fleet insurance is evolving at a remarkable pace, driven by technological advancements and the urgent need for more effective risk management strategies in the commercial auto sector. With rising operational costs and increasing safety concerns, fleet managers are seeking solutions that not only streamline their operations but also enhance their ability to mitigate risks. Enter Full Stack AI—a transformative technology that plays a crucial role in redefining how fleet insurance and risk management are approached.
Full Stack AI encompasses a comprehensive approach by integrating various AI capabilities into a cohesive framework. By leveraging data from diverse sources, it empowers fleet managers with actionable insights and automation advantages that simplify decision-making processes. This new breed of artificial intelligence is uniquely positioned to address many challenges faced in the commercial fleet space, including risk assessment, claims handling, and fraud detection.
What is Full Stack AI and How Does it Enhance Fleet Insurance?
Defining Full Stack AI
Full Stack AI refers to a complete framework that combines multiple layers of artificial intelligence to generate complex insights from large datasets. This integrated approach facilitates the seamless functioning of various AI components, enabling fleet managers to access real-time information and analytics. Full Stack AI differs from traditional AI applications by offering end-to-end solutions that operationalize data across diverse channels and workflows, removing silos and enhancing collaboration.
Key Components of Full Stack AI in Insurance
The architecture of Full Stack AI is built upon several key components that contribute to its effectiveness in fleet insurance. These include:
- Data Collection: Utilizing telematics devices and AI-driven data acquisition methods to gather information on vehicle performance and driver behavior.
- Data Processing: Employing advanced algorithms for data cleansing, normalization, and integration to ensure accuracy and reliability.
- Analytics and Insights: Analyzing data to extract predictive insights that inform risk assessments, operational decisions, and strategic planning.
- Automation: Facilitating streamlined workflows that enhance claims processing and underwriting through machine learning and AI automation technologies.
Benefits of a Full Stack Approach for Fleet Management
The implementation of Full Stack AI in fleet management offers a variety of significant benefits, such as improved accuracy in risk assessments, enhanced claims processing accuracy, and the ability to swiftly analyze and respond to changing conditions. By utilizing data from a wide array of sources—including telematics, driving behavior analytics, and market trends—fleet managers can develop a holistic understanding of potential risks. This promotes informed, strategic decision-making.
How Does Full Stack AI Optimize Risk Assessment in Fleet Insurance?
The Role of AI Telematics in Data Collection
AI telematics systems are at the forefront of data collection in fleet insurance. By equipping vehicles with telematics devices, fleet operators can monitor real-time information regarding vehicle location, speed, maintenance needs, and driver behaviors. This continuous flow of data allows for timely interventions and proactive risk management strategies, such as identifying unsafe driving patterns before they lead to accidents or insurance claims.
Commercial Auto Analytics: Transforming Raw Data into Insights
Turning raw data collected from telematics into actionable insights relies heavily on commercial auto analytics. This segment of Full Stack AI applies advanced statistical and machine learning models to analyze historical data, identify trends, and map out potential risk scenarios. By synthesizing complex datasets, fleet managers are better equipped to understand how various factors influence driver safety, vehicle maintenance, and overall fleet performance.
Predictive Modeling for Improved Risk Profiles
Predictive modeling within Full Stack AI enhances risk profiling by forecasting potential incidents based on historical data. For instance, machine learning algorithms can identify patterns that precede accidents or insurance claims, enabling fleet managers to implement preventative measures tailored to specific risk factors. This forward-thinking approach minimizes uncertainties, allowing businesses to allocate resources more effectively and sustainably manage their insurance portfolios.
In What Ways Does Full Stack AI Improve Claims Accuracy?
Streamlining Claims Processing through Automation
The convening of claims management and Full Stack AI leads to a streamlined process flow that enhances accuracy and efficiency. What once took days or weeks to assess can be reduced to hours through automated workflows that handle data ingestion, verification, and assessment in real time. By harnessing intelligent automation, fleet insurers significantly reduce manual errors and fast-track claims resolutions.
AI-Driven Claims Adjudication: Speed and Precision
Full Stack AI facilitates precise claims adjudication powered by machine learning algorithms. These systems analyze various elements of a claim, assessing factors such as incident reports, witness statements, and vehicle performance data to deliver accurate decisions based on available evidence. The speed and precision offered by AI-driven adjudication not only improves customer satisfaction but also strengthens the integrity of the insurance process.
Real-time Data Integration for Accurate Valuation
One of the most significant advantages that Full Stack AI brings to claims processing is real-time data integration. By automatically cross-referencing multiple data points, insurers can establish accurate valuations on claims, leading to fair settlements. This capability minimizes discrepancies caused by human error and provides a robust framework for resolving disputes quickly, enabling fleet managers to maintain trust within their operations.
How Can Full Stack AI Prevent Fraud in Fleet Insurance?
Identifying Red Flags with Advanced Analytics
Fraud prevention is a critical aspect of fleet insurance, and Full Stack AI excels in this domain through advanced analytics. By analyzing claims data against historical trends, AI systems can detect anomalies and red flags that signify potential fraudulent activity. Employing these sophisticated analytical techniques significantly reduces the risk of financial losses attributed to fraudulent claims.
Machine Learning Algorithms for Fraud Detection
Machine learning algorithms automatically learn and adapt based on new data inputs, empowering insurers to improve their fraud detection capabilities. As more data is processed, these algorithms continuously refine their criteria for identifying suspicious claims, enhancing accuracy and responsiveness. This iterative learning process provides fleet managers with a proactive tool to combat fraud, ultimately saving costs associated with fraudulent claims processing.
Case Studies: Successful Fraud Prevention in the Field
Successful implementations of Full Stack AI have demonstrated how effective data analysis can prevent fraud in fleet insurance. Companies utilizing AI-driven predictive analytics have reported significant reductions in fraudulent claims, showcasing the power of modern technology in safeguarding financial resources and ensuring the integrity of insurance operations. The ability to identify and mitigate risk beforehand fosters trust and security within the fleet management ecosystem.
What is the Impact of Driver Behavior Tracking on Fleet Safety?
The Importance of Monitoring Driver Performance
Monitoring driver performance is vital for enhancing fleet safety and reducing risks. Full Stack AI enables fleet managers to gather data on a driver's habits, such as braking patterns, acceleration, and adherence to speed limits. This vital information supports both individual driver evaluations and overall fleet assessments, allowing for targeted interventions when necessary.
Behavioral Analytics for Risk Mitigation
Behavioral analytics derived from driver performance data provide actionable insights that can mitigate risks. For instance, by understanding which drivers exhibit dangerous behaviors, fleet managers can implement training programs aimed at improving driver safety. Furthermore, insights from this data can reveal broader trends that inform company policies and operational goals, driving safety standards across the entire fleet.
Training and Incentive Programs Based on Analytics
Incorporating analytics into training and incentive programs creates an environment focused on continuous improvement. Fleet managers can design programs that reward safe driving practices or help drivers develop skills based on their unique performance metrics. This strategic approach not only fosters a culture of safety but also translates into lower insurance costs and fewer accidents.
How Can Fleet Managers Leverage Full Stack AI for Strategic Decision Making?
Data-Driven Insights for Resource Allocation
Leveraging Full Stack AI empowers fleet managers to make more informed decisions regarding resource allocation. The insights generated from real-time data allow for greater efficiency in deploying vehicles, managing fuel consumption, and optimizing routes. This data-driven approach ensures that fleet resources are utilized effectively, ultimately improving operational performance.
Utilizing AI for Fleet Optimization and Efficiency
AI-driven optimization models enable fleet managers to continuously assess vehicle and driver performance, leading to enhancements in overall efficiency. Full Stack AI facilitates the identification of maintenance needs, optimal scheduling, and traffic pattern predictions, reducing downtime and operational costs. These advancements are instrumental in achieving strategic business goals while maintaining a competitive edge in the market.
Long-Term Planning with Predictive Analytics
Furthermore, predictive analytics offer fleet managers valuable foresight into long-term planning. By examining trends over time and projecting future risks, fleet managers can develop strategic initiatives that respond to anticipated challenges. These insights inform insurance negotiations, budget planning, and investment decisions to ensure that the fleet remains agile and adaptable in an ever-changing commercial landscape.
Conclusion
In conclusion, the transformative effects of Full Stack AI on fleet insurance and risk management are evident in its operational efficiency, enhanced risk assessment capabilities, and proactive fraud prevention strategies. These technologies provide the framework necessary for fleet managers to navigate the complexities of the commercial auto sector while minimizing risks and maximizing profitability. As advancements in AI technology continue to unfold, fleet managers are encouraged to explore the integration of Full Stack AI into their practices.
To learn more about the innovative solutions Inaza offers, including our state-of-the-art insights via the Decoder platform, contact us today or book a demo to see how Full Stack AI can revolutionize your fleet insurance and risk management strategies.